Introduction
In the rapidly evolving digital age, data is often considered the new oil. This is particularly true in sectors that rely heavily on data for insights and innovation, such as artificial intelligence (AI) and machine learning (ML). The backbone of successful AI and ML applications is high-quality data, meticulously annotated and curated. However, collecting and annotating this data is no small feat, especially for businesses seeking to harness the power of AI without dedicating excessive resources to data management. This is where lead data collection companies come into play.
Choosing the right lead data collection company for annotation can be a daunting task, given the myriad of options available. This guide aims to simplify this process by exploring key considerations, and highlighting top companies in the field.
Understanding Data Annotation and Its Importance
What is Data Annotation?
Data annotation is the process of labeling data to make it understandable for AI and ML models. This can involve tagging images, transcribing audio, labeling text, and more. The quality of these annotations directly impacts the performance of AI models. Poorly annotated data can lead to inaccurate predictions, while well-annotated data can significantly enhance model accuracy and reliability.
Why is Data Annotation Important?
- Improves Model Accuracy: High-quality annotated data enables AI models to learn patterns and make predictions with greater accuracy.
- Facilitates Complex Analysis: Annotated data allows for the analysis of complex relationships within the data, which is crucial for advanced applications like natural language processing (NLP) and computer vision.
- Enhances Training Efficiency: Properly labeled data reduces the time required to train AI models, accelerating the development process.
- Ensures Compliance: In regulated industries such as healthcare and finance, annotated data helps ensure compliance with legal and ethical standards.
Key Factors to Consider When Choosing a Data Annotation Company
Selecting the right data annotation company involves evaluating several critical factors. Here’s what to consider:
1. Quality of Annotation
The primary factor in choosing a data annotation company is the quality of their annotations. High-quality annotations ensure that AI models are trained on accurate and reliable data. Look for companies that employ rigorous quality control measures, such as multiple review layers, consistency checks, and expert oversight.
Example: A company with a detailed quality assurance process might use a combination of automated tools and human reviewers to validate annotations, ensuring high accuracy.
2. Scalability
Data projects can vary significantly in size and complexity. It’s essential to choose a company that can scale its services to match your project’s requirements. Whether you need annotations for a few hundred images or several million data points, the company should be able to handle your needs efficiently.
Example: Companies with a large workforce and flexible resources can quickly adapt to changing project sizes, providing the necessary support as your data requirements grow.
3. Turnaround Time
In fast-paced industries, timely delivery of annotated data is crucial. Ensure that the data annotation company can meet your project deadlines without compromising quality. A company’s ability to provide quick turnaround times can be a significant advantage, especially for projects with tight schedules.
Example: Companies that use advanced technologies and streamlined processes are often better equipped to deliver annotated data swiftly.
4. Cost
Budget considerations are always important. While it’s crucial not to compromise on quality, finding a company that offers competitive pricing is essential. Look for companies that provide transparent pricing models and can tailor their services to fit your budget.
Example: Some companies offer flexible pricing based on the volume of data or the complexity of the annotation task, allowing you to manage costs effectively.
5. Technology and Tools
Advanced technology and tools can enhance the efficiency and accuracy of data annotation. Look for companies that utilize cutting-edge software, machine learning algorithms, and automation to support human annotators and improve overall performance.
Example: Companies that integrate AI-driven tools for pre-labeling or data management can expedite the annotation process and reduce errors.
6. Experience and Expertise
The experience and expertise of the data annotation company play a significant role in the quality of the output. Companies with a proven track record and domain-specific knowledge are better equipped to handle complex and specialized data annotation tasks.
Example: A company with extensive experience in annotating medical images will have a deeper understanding of the nuances and requirements specific to the healthcare industry.
7. Data Security
Data security is paramount, especially when dealing with sensitive or proprietary information. Ensure that the data annotation company has robust security measures in place, including encryption, secure data handling protocols, and compliance with data protection regulations.
Example: Companies that adhere to industry standards for data security, such as GDPR or HIPAA compliance, provide an added layer of protection for your data.
8. Cultural and Linguistic Diversity
For projects involving global data, cultural and linguistic diversity among annotators can enhance the quality of annotations. Companies with a diverse workforce can provide more accurate and contextually relevant annotations for multilingual and multicultural data.
Example: Companies with a global network of annotators can handle diverse datasets and provide insights that might be missed by a more homogenous team.
Top Lead Data Collection Companies for Annotation
Let’s explore the leading data collection service providers, highlighting their offerings, technological innovations, industry applications, and customer-centric approaches.
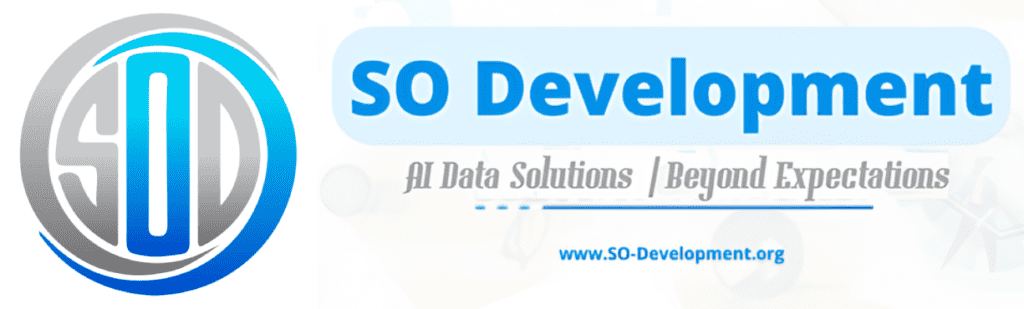
SO Development:
Overview: SO Development, or Sustainable and Organic Development, emphasizes long-term growth, sustainability, and ethical practices. When choosing a data annotation partner, it’s essential to consider how their services align with these principles:
Strengths:
- Advanced tools
- Expert team
- Flexibility
- Rigorous quality control
Why It Stands Out: SO Development prioritizes client satisfaction through proactive project management, continuous improvement in annotation techniques, and responsive customer support, fostering long-term partnerships and successful AI implementations.
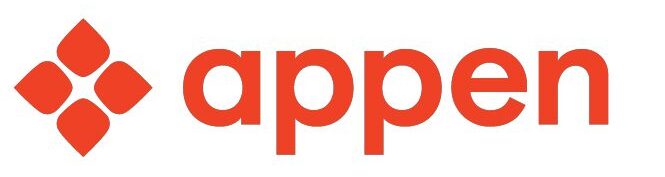
Appen
Overview: Appen is a leader in the data annotation industry, known for its extensive global crowd of over a million workers. They offer a broad range of annotation services, including text, image, video, and audio data.
Strengths:
- Diverse talent pool
- Scalable solutions
- Rigorous quality control
- Advanced technology integration
Why It Stands Out: Appen’s global reach and diverse workforce enable them to provide high-quality annotations for a wide range of data types. Their scalability and experience make them a reliable partner for both small and large projects.

Lionbridge AI
Overview: Lionbridge AI, a division of Lionbridge Technologies, specializes in AI training data and annotation services. They offer comprehensive solutions tailored to various industries, including technology, finance, and healthcare.
Strengths:
- Industry expertise
- Multilingual capabilities
- Quality assurance
- Global workforce
Why It Stands Out: Lionbridge AI’s deep understanding of AI and machine learning, combined with their multilingual and multi-regional capabilities, make them a strong choice for diverse and complex data annotation projects.
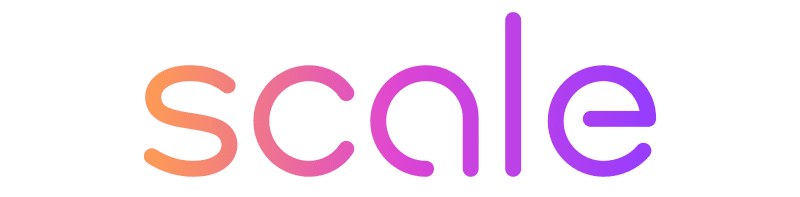
Scale AI
Overview: Scale AI uses a technology-driven approach to data annotation, combining human expertise with machine learning to enhance efficiency and accuracy. Their platform is designed to handle complex data tasks with speed and precision.
Strengths:
- Technological innovation
- Customization
- Rapid turnaround
- Hybrid model (human + machine)
Why It Stands Out: Scale AI’s integration of human and machine capabilities allows for efficient and accurate data annotation, making them ideal for projects requiring both speed and quality.
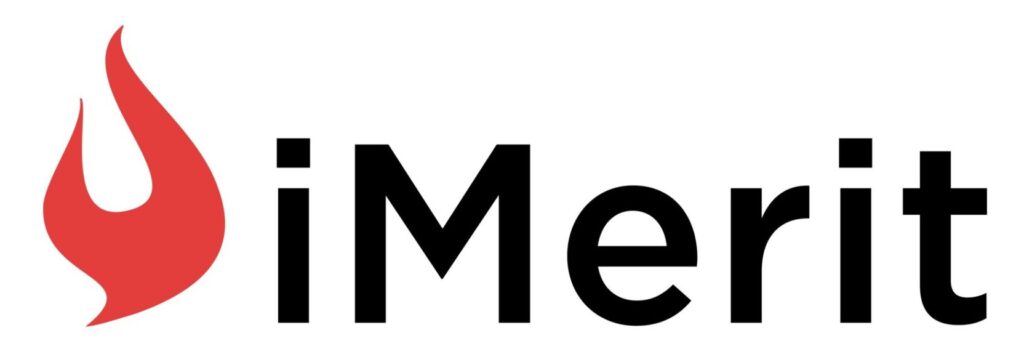
iMerit
Overview: iMerit provides high-quality data annotation and enrichment services across various industries, with a strong emphasis on accuracy and scalability. They offer tailored solutions to meet the specific needs of their clients.
Strengths:
- Industry-specific expertise
- Robust quality control
- Scalable solutions
- Experienced team
Why It Stands Out: iMerit’s commitment to industry-specific solutions and detailed quality assurance processes makes them a preferred choice for businesses seeking reliable and precise data annotation.
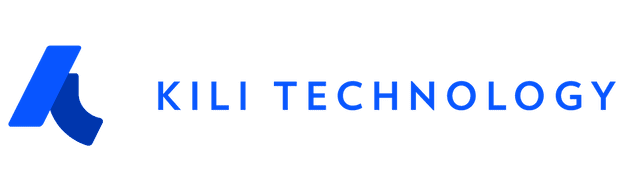
Kili Technology
Overview: Kili Technology offers a comprehensive data annotation platform designed to streamline the labeling process and enhance productivity. Their platform provides tools for efficient data management and annotation.
Strengths:
- Platform efficiency
- User-friendly interface
- Built-in quality control
- Advanced tools
Why It Stands Out: Kili Technology’s platform-centric approach provides a complete and efficient solution for managing and annotating large datasets, improving productivity and accuracy.
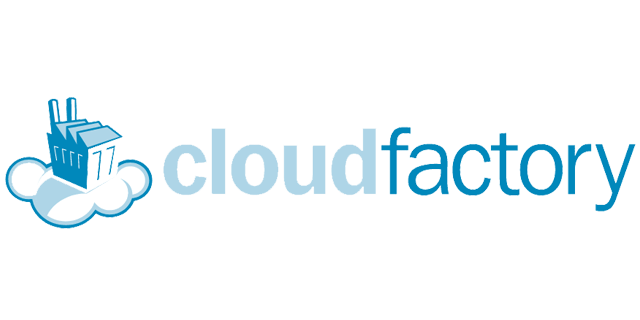
CloudFactory
Overview: CloudFactory combines human intelligence with automation to deliver efficient and accurate data annotation services. Their global workforce and advanced technology enable them to handle diverse and large-scale projects.
Strengths:
- Hybrid model (human + automation)
- Global reach
- Industry focus
- Efficient processes
Why It Stands Out: CloudFactory’s hybrid approach allows for efficient and accurate annotation, catering to various industries and providing a balance between speed and quality.
Best Practices for Collaborating with Data Annotation Companies
To maximize the benefits of working with a data annotation company, consider the following best practices:
1. Define Clear Objectives
Before starting a project, clearly define your objectives and requirements. Provide detailed instructions and guidelines to the data annotation company to ensure that the annotations meet your specific needs.
Example: Prepare a comprehensive project brief that outlines the goals, data types, and annotation requirements to ensure alignment between your expectations and the company’s deliverables.
2. Communicate Effectively
Maintain open and effective communication with the data annotation company throughout the project. Regular updates, feedback, and discussions can help address any issues promptly and ensure that the project stays on track.
Example: Schedule regular check-ins or status meetings to review progress, address concerns, and provide feedback on the quality of annotations.
3. Leverage Technology
Take advantage of the company’s technological capabilities to enhance the efficiency and accuracy of the annotation process. Utilize platforms, tools, and automation features that can streamline workflows and improve results.
Example: Use annotation platforms that offer advanced features such as real-time collaboration, integrated quality checks, and data management tools.
4. Monitor Quality
Implement a system for monitoring and evaluating the quality of annotations. Regularly review samples of annotated data to ensure that it meets your standards and make adjustments as needed.
Example: Perform periodic quality audits or reviews of annotated data to identify and address any discrepancies or issues.
5. Build a Collaborative Relationship
Foster a collaborative relationship with the data annotation company to create a productive working environment. Establishing trust and collaboration can lead to better outcomes and a more successful partnership.
Example: Engage in collaborative problem-solving and work together to overcome challenges, ensuring that both parties are aligned towards achieving project goals.
Conclusion
Choosing the best lead data collection companies for data annotation is a critical decision that impacts the success of AI and machine learning projects. By considering factors such as quality, scalability, turnaround time, cost, technology, experience, data security, and cultural diversity, you can find a partner that meets your specific needs.
Top data annotation companies like Appen, Lionbridge AI, Sama, Scale AI, iMerit, CloudFactory, Tejas Data, Kili Technology, DataLab, and Scribe offer a range of solutions and strengths to suit various projects. Aligning your choices with SO Development principles ensures that your data annotation efforts are ethical, sustainable, and conducive to long-term success.
By following best practices for collaboration and leveraging technology, you can maximize the effectiveness of your data annotation projects and contribute to the advancement of AI and machine learning in a responsible and impactful way.