Introduction
In today’s digital age, data is the lifeblood of businesses. The sheer volume of information generated every second offers untapped opportunities for companies to innovate, adapt, and remain competitive in their respective industries. But raw data alone isn’t enough. To transform data into actionable insights, companies need to organize, structure, and contextualize it—a process known as data annotation. As Artificial Intelligence (AI) and Machine Learning (ML) become pivotal for growth, data annotation emerges as a crucial foundation for building advanced systems that can help companies stay ahead of the curve.
This blog will explore how data annotation fuels innovation, efficiency, and competitiveness, examining the techniques, benefits, challenges, and real-world examples that demonstrate its significance.
Understanding Data Annotation
Data annotation refers to the process of labeling data to make it understandable for machine learning models. Whether it’s identifying objects in an image, categorizing text sentiment, or tagging voice data with emotions, annotated data helps machines recognize patterns, make decisions, and continuously improve their performance. Without annotation, ML models are blind; they need this contextualized data to learn and produce accurate results.
Types of Data Annotation:
- Text Annotation: Labeling text data with information like sentiment, intent, entities (e.g., names, places), or categorizing documents for tasks like natural language processing (NLP).
- Image Annotation: Identifying objects, bounding boxes, and segmenting different areas of an image, used in applications like facial recognition, medical imaging, and self-driving cars.
- Audio Annotation: Tagging voice data with attributes like emotions, speaker identification, or transcription for voice assistants, call centers, and more.
- Video Annotation: Similar to image annotation but adds a temporal element—tracking moving objects over time, useful in areas like surveillance, sports analytics, or autonomous vehicles.
The Role of Data Annotation in AI and Machine Learning
For AI and machine learning models to function effectively, they need annotated datasets that teach them to recognize patterns, interpret results, and generalize beyond their training data. Whether it’s chatbots understanding human language or robots navigating their environments, annotated data is the fuel that powers these innovations.
As companies strive to stay competitive, AI-driven solutions like predictive analytics, automation, and personalized experiences are becoming essential. However, building AI solutions without the right training data is like teaching someone to drive without access to roads—there’s no direction or context. Data annotation provides this much-needed context, enabling businesses to develop AI systems that deliver value.
AI Innovations Powered by Data Annotation:
- Self-driving Cars: Advanced driver-assistance systems (ADAS) rely on annotated data to recognize traffic signs, pedestrians, and other vehicles.
- Healthcare Diagnostics: AI models trained on annotated medical images can assist doctors in diagnosing diseases like cancer or detecting anomalies in X-rays and MRIs.
- E-commerce Personalization: Recommendation engines depend on annotated user behavior and product data to suggest relevant items to shoppers.
- Voice Assistants: Systems like Alexa and Siri understand and respond to voice commands because they are trained on vast datasets of annotated audio.
Competitive Advantages of Data Annotation
Companies investing in data annotation gain several advantages that enhance their competitive edge, both operationally and strategically. The use of well-annotated data allows businesses to optimize their decision-making, offer innovative services, and better understand customer needs.
1. Improved Decision-Making
Data annotation ensures that AI systems are accurate and reliable, leading to better decision-making. In industries like finance, AI models can detect fraud patterns, assess risks, and predict market trends when trained on annotated datasets. Accurate insights into these areas can help companies make swift, informed decisions that outpace competitors.
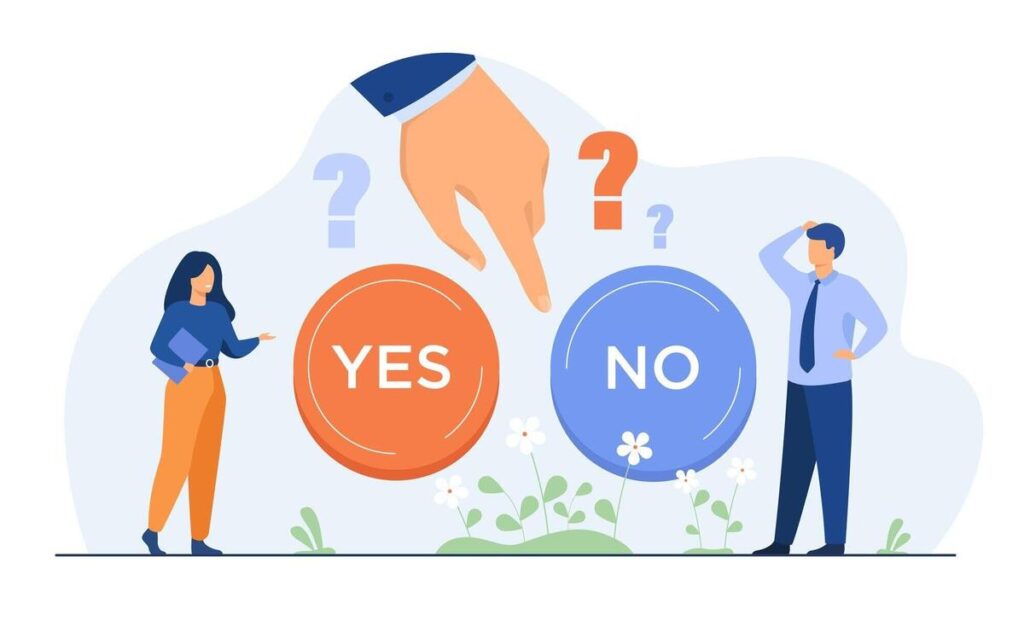
2. Enhanced Customer Experience
Personalization is no longer just a nice-to-have feature—it’s an expectation. Data annotation allows companies to better understand their customers’ preferences, behaviors, and needs. AI models that predict what customers want can provide personalized product recommendations, targeted marketing messages, or tailored customer support. A company that can anticipate and cater to individual customer needs will outshine competitors that provide generic experiences.
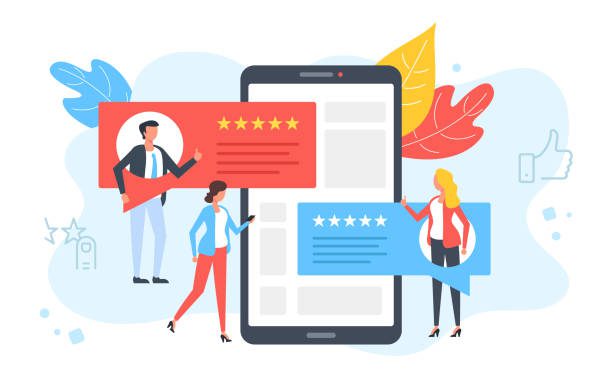
3. Operational Efficiency
Efficiency is key to staying competitive. Companies that use AI models built on well-annotated data can automate repetitive tasks, streamline processes, and reduce human error. For instance, in logistics, AI systems can optimize delivery routes, inventory management, and demand forecasting, all of which contribute to faster, more efficient operations.
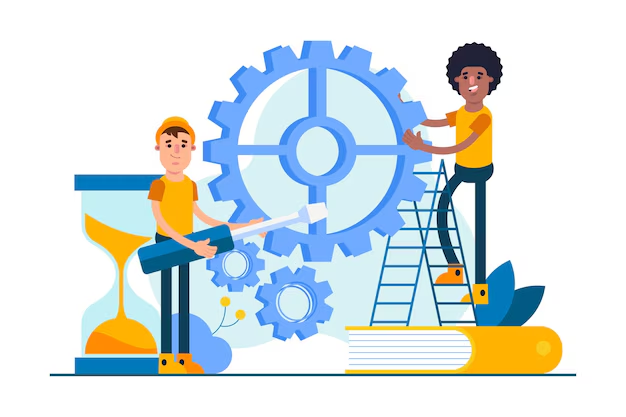
4. Innovation and Product Development
Data annotation fuels innovation by enabling companies to develop new products and services. For example, a retail company can use annotated image data to create virtual fitting rooms that allow customers to “try on” clothes using augmented reality. Similarly, in the automotive industry, annotated data powers the development of autonomous vehicles.
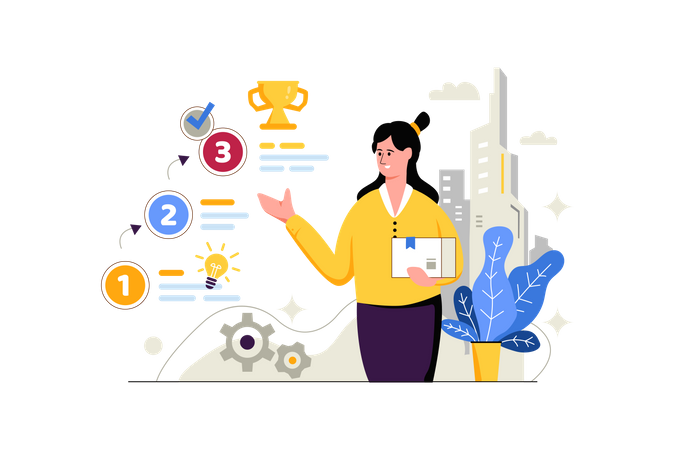
Challenges in Data Annotation
While the benefits of data annotation are clear, the process itself is not without challenges. Annotating large datasets requires time, resources, and expertise. Companies that fail to invest in high-quality data annotation may struggle to build effective AI models, resulting in inaccurate predictions, poor performance, and ultimately, loss of competitive advantage.
1. Cost and Resource Intensiveness
Data annotation is a labor-intensive process that can be expensive, especially for companies that need to label large datasets. Hiring human annotators, managing annotation workflows, and ensuring quality control all add to the cost.
2. Data Privacy and Security
In industries like healthcare or finance, where data sensitivity is a major concern, maintaining privacy during the annotation process is critical. Ensuring that annotators only have access to de-identified or anonymized data is necessary to protect customer information and comply with regulations like GDPR.
3. Scalability
As businesses grow and data volumes increase, scaling the annotation process can be challenging. Manual annotation processes that work for small datasets may not be sustainable for larger ones. Companies need to invest in automation or crowdsourcing solutions to keep up with growing annotation needs.
4. Annotation Quality and Consistency
The accuracy of a machine learning model directly depends on the quality of the annotated data. Poor or inconsistent annotations can lead to incorrect predictions, undermining the entire AI system. Ensuring quality control through rigorous annotation guidelines, training for annotators, and periodic reviews is essential.
Best Practices for Effective Data Annotation
To ensure that companies can maximize the benefits of data annotation, there are several best practices they can follow. These include a combination of human-in-the-loop (HITL) approaches, leveraging technology, and focusing on data quality and security.
1. Human-in-the-Loop (HITL)
While automation can assist with some aspects of data annotation, human oversight is crucial for maintaining accuracy. In a HITL model, humans and machines collaborate to ensure high-quality annotations. Machines can handle the repetitive tasks, while humans can focus on reviewing complex or ambiguous cases.
2. Leveraging AI-Assisted Tools
AI itself can be used to streamline the annotation process. Tools that use machine learning algorithms can pre-label data, which can then be fine-tuned by human annotators. This significantly speeds up the annotation process and reduces costs while still maintaining quality.
3. Crowdsourcing Solutions
For companies with large datasets, crowdsourcing platforms like Amazon Mechanical Turk or specialized data annotation services provide access to a vast pool of annotators. This approach allows businesses to scale quickly while maintaining flexibility in the annotation process.
4. Quality Control Mechanisms
To ensure consistent and accurate annotations, companies should implement quality control mechanisms such as inter-annotator agreement metrics, regular audits, and feedback loops. Annotators should be trained, and their performance should be regularly evaluated to maintain high standards.
Real-World Case Studies: Companies Thriving with Data Annotation
1. Tesla: Pioneering Self-Driving with Annotated Data
Tesla’s AI-driven self-driving cars rely heavily on annotated data for training. Every car on the road captures millions of images and videos, which are then annotated by Tesla’s team to teach the AI system how to recognize traffic signs, pedestrians, other vehicles, and road conditions. This annotated data allows Tesla to continuously improve its autonomous driving software, giving it a significant edge over competitors in the electric vehicle market.
2. Google: Enhancing Search Algorithms with Text and Image Annotation
Google’s search algorithms rely on a vast dataset of annotated text and images. By labeling content with context like relevance, subject matter, and sentiment, Google can deliver more accurate search results and personalized recommendations. Additionally, Google uses annotated data to power its Google Lens feature, which allows users to search the web by taking a photo.
3. Amazon: Optimizing Customer Experience with Personalized Recommendations
Amazon’s recommendation engine is one of the key factors behind its success. By using annotated customer behavior data—such as purchase history, browsing activity, and product reviews—Amazon can offer highly personalized product suggestions to its users. This tailored shopping experience keeps customers engaged and increases sales, helping Amazon stay ahead of its competitors.
4. Apple: Elevating Voice Assistants with Audio Annotation
Apple’s Siri is trained on vast amounts of annotated voice data. Audio annotations, which include labeling speech patterns, accents, and emotional tones, help Siri understand and respond to a wide range of user commands. The continuous improvement in Siri’s natural language processing capabilities is driven by annotated datasets, giving Apple a competitive edge in the voice assistant market.
Future of Data Annotation: Trends and Opportunities
As companies continue to push the boundaries of AI and machine learning, data annotation will only grow in importance. Several trends and opportunities are shaping
the future of this space.
1. Automated Annotation
The rise of AI-driven annotation tools promises to reduce the manual burden of labeling data. These tools, powered by ML algorithms, can autonomously label data and improve over time with feedback. Companies that invest in automated annotation can scale their AI initiatives faster while reducing costs.
2. Specialized Annotation Services
As AI becomes more specialized, the need for domain-specific annotation will increase. For example, medical AI systems will require annotators with healthcare expertise to label complex datasets like MRI scans or pathology reports. Companies that offer specialized annotation services will become indispensable to businesses in highly regulated or technical industries.
3. Data Anonymization and Privacy-Centric Annotation
With data privacy regulations tightening across the globe, companies will need to ensure that their annotation processes comply with laws like GDPR and CCPA. The future will see a growing demand for privacy-centric annotation solutions, where sensitive data is anonymized or de-identified before being labeled.
4. Crowdsourced Data Annotation at Scale
Crowdsourcing will continue to play a key role in data annotation. As companies gather more data than ever before, they will need large-scale, flexible annotation solutions. Crowdsourcing platforms can provide the necessary scalability, allowing companies to annotate massive datasets quickly without sacrificing quality.
Conclusion
In the fast-paced world of technology and innovation, data annotation is a critical component that helps companies stay competitive. It forms the backbone of AI and machine learning systems, providing the context necessary for machines to learn, adapt, and improve. By investing in high-quality data annotation, companies can unlock the full potential of AI, streamline operations, enhance customer experiences, and foster continuous innovation. As industries continue to evolve, those who prioritize data annotation will lead the charge, outpacing competitors and driving lasting success.
The future is bright for companies that recognize the power of data annotation, and as the technology matures, the opportunities for growth, innovation, and competitiveness will only expand further.