Introduction
Generative Artificial Intelligence (Generative AI) is a cutting-edge technology that has revolutionized the landscape of artificial intelligence. Unlike traditional AI models that are designed for specific tasks, generative AI has the remarkable ability to create new content, whether it be images, text, or even music. In this comprehensive article, we delve into the world of generative AI, exploring its underlying principles, applications across diverse industries, ethical considerations, and the potential it holds for shaping the future of innovation.
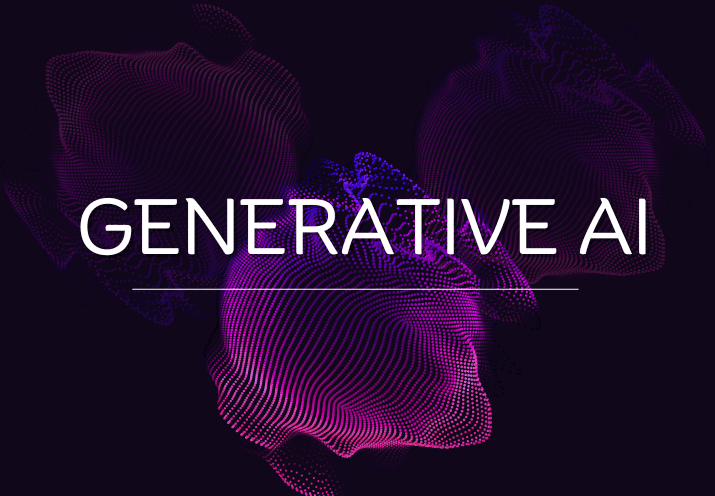
1.Understanding Generative AI
1.1 Defining Generative AI
Generative AI refers to a class of artificial intelligence algorithms designed to generate new, unique content autonomously. Unlike other AI models that are task-specific and rely on pre-existing data, generative AI has the capacity to create content that has not been explicitly programmed or seen during training.
1.2 Evolution of Generative AI
The development of generative AI can be traced back to the early days of neural networks and machine learning. Over time, advancements in deep learning, particularly the introduction of Generative Adversarial Networks (GANs) and Variational Autoencoders (VAEs), have propelled generative AI into the forefront of AI research and application.
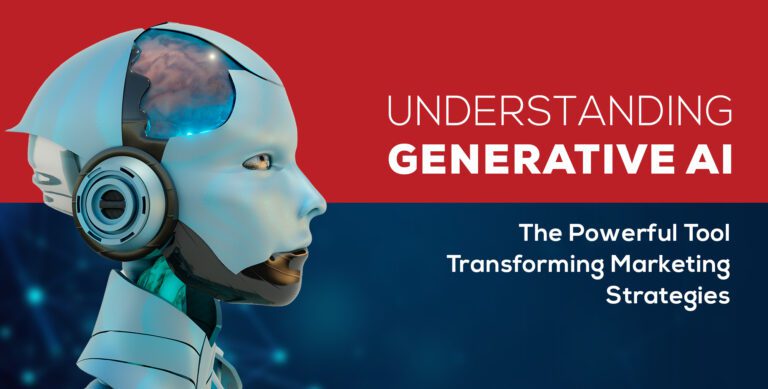
2. Key Components of Generative AI
2.1 Neural Networks
Generative AI relies heavily on neural networks, specifically deep neural networks. These networks, inspired by the human brain’s structure, consist of interconnected layers of artificial neurons that process and analyze data to generate new content.
2.2 Generative Adversarial Networks (GANs)
GANs are a fundamental architecture within generative AI. Consisting of two neural networks—the generator and the discriminator—GANs work in tandem, with the generator creating content and the discriminator evaluating its authenticity. This adversarial training process results in the continuous improvement of generated content.
2.3 Variational Autoencoders (VAEs)
VAEs are another key architecture in generative AI. They are designed to learn the underlying distribution of the input data and generate new content by sampling from this distribution. VAEs are particularly effective in generating diverse and novel outputs.
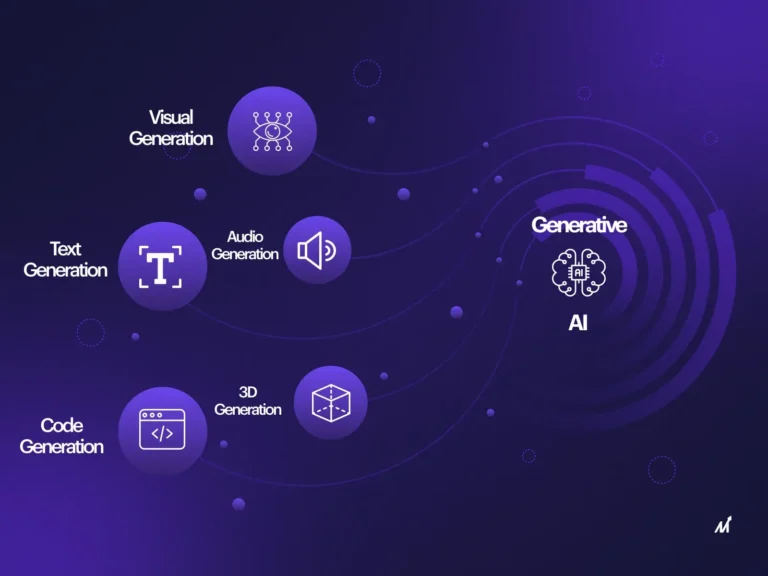
3. Applications of Generative AI
3.1 Image Generation and Style Transfer
Generative AI has demonstrated remarkable success in generating realistic and high-quality images. Style transfer applications allow users to apply the artistic style of one image to another, fostering creativity and enabling the creation of unique visual content.
3.2 Natural Language Processing
In the realm of natural language processing, generative AI has found applications in text generation, chatbots, and even the creation of coherent and contextually relevant paragraphs. OpenAI’s GPT (Generative Pre-trained Transformer) models are exemplary in this domain.
3.3 Creative Arts and Design
Generative AI has become a powerful tool for artists and designers. From generating original artworks to creating novel design concepts, generative AI has opened up new avenues for creative expression and collaboration between human and machine.
3.4 Music Composition
The ability of generative AI to understand and mimic patterns extends to the realm of music. AI algorithms can compose original music, mimicking the style of specific genres or even blending diverse musical influences to create entirely new compositions.
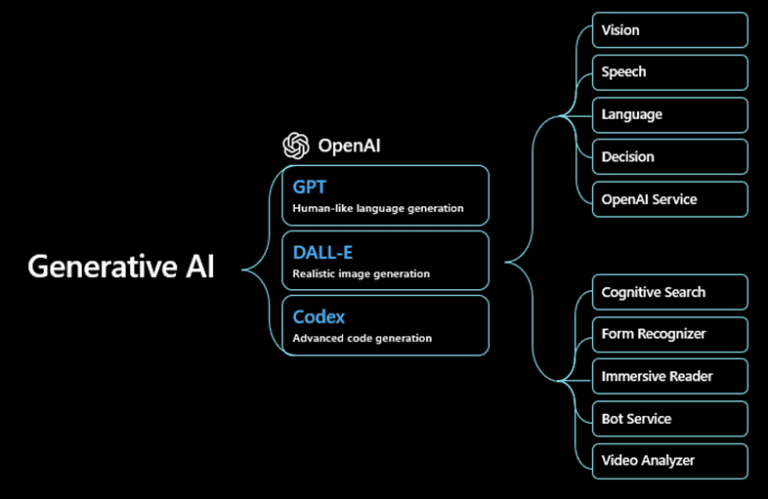
4. Generative AI in Healthcare
4.1 Drug Discovery
Generative AI is accelerating drug discovery by predicting molecular structures that have the potential to become new drugs. This streamlines the research process, reducing the time and resources required for drug development.
4.2 Medical Imaging
In medical imaging, generative AI can enhance image resolution, generate synthetic images for training purposes, and assist in the early detection of anomalies. This has profound implications for improving diagnostic accuracy and patient outcomes.
4.3 Personalized Medicine
Generative AI analyzes patient data to create personalized treatment plans based on an individual’s unique genetic makeup. This approach holds the promise of tailoring medical interventions to the specific needs of each patient.
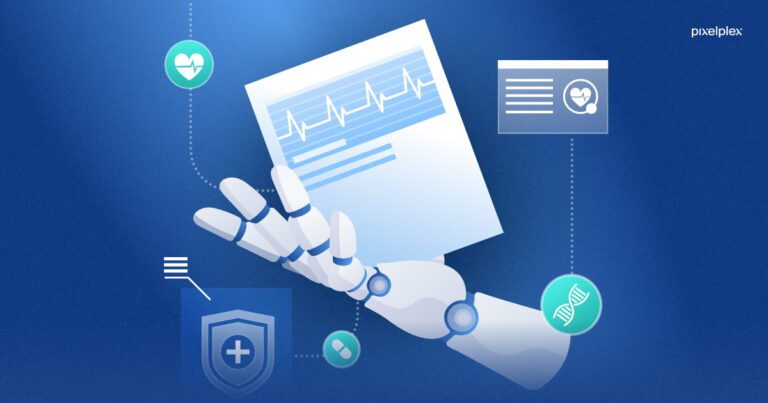
5. Ethical Considerations and Challenges
5.1 Bias in Generative AI
Generative AI models are not immune to biases present in training data. Ensuring that generated content is free from biases and adheres to ethical standards is a critical challenge that the AI community must address.
5.2 Deepfakes and Misuse
The ability of generative AI to create highly realistic content raises concerns about the potential for misuse, particularly in the creation of deepfakes—manipulated videos that can convincingly depict individuals saying or doing things they never did. Addressing these ethical concerns is imperative.
5.3 Security and Privacy
Generative AI‘s capacity to create realistic images and content has implications for security and privacy. Safeguarding against malicious use, such as creating synthetic identities or generating realistic but fake documentation, is a growing concern.
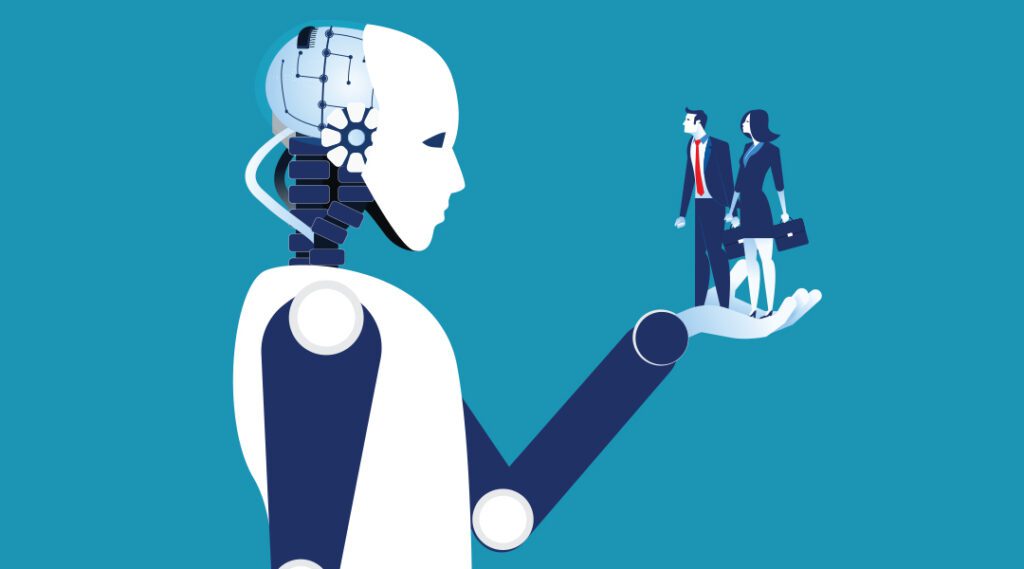
6. The Future of Generative AI
6.1 Advances in Model Architectures
The future of generative AI will likely see continuous advancements in model architectures, leading to more sophisticated and capable algorithms. Researchers are exploring novel architectures that can handle a broader range of tasks and generate even more realistic content.
6.2 Human-AI Collaboration
Generative AI is expected to foster increased collaboration between humans and machines, especially in creative fields. Human-AI partnerships may lead to the co-creation of innovative solutions in art, design, and other domains.
6.3 Improved Interpretability
As generative AI becomes more prevalent, efforts to enhance the interpretability of AI-generated content will be crucial. Understanding how and why a model generates specific outputs will be essential for building trust and ensuring responsible use.
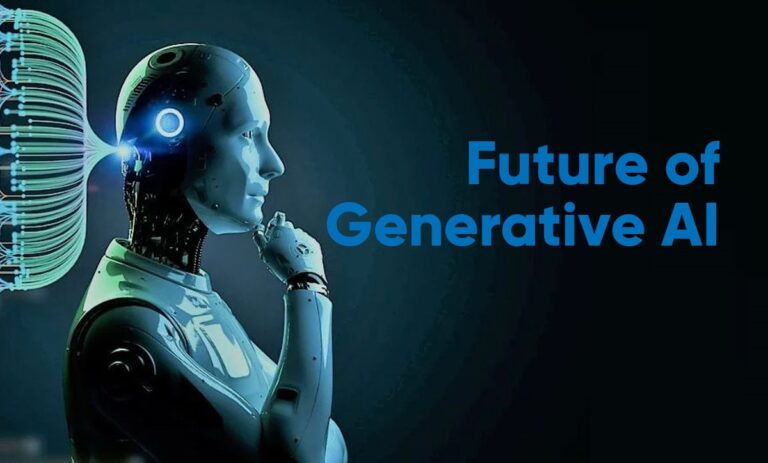
Conclusion
Generative AI represents a paradigm shift in artificial intelligence, unlocking new possibilities across diverse industries. From creating art to advancing healthcare, generative AI has the potential to reshape the way we live, work, and interact with technology. While ethical considerations and challenges must be addressed, the future of generative AI holds immense promise for fostering creativity, innovation, and collaboration between humans and intelligent machines.