1. Introduction
Artificial Intelligence (AI) has revolutionized the world in more ways than one. From healthcare and finance to entertainment and transportation, AI has made its presence felt across a spectrum of industries. However, one particular area that has garnered significant attention and reshaped how we perceive AI’s creative capabilities is Generative Adversarial Networks (GANs). GANs have rapidly evolved to become a pivotal part of AI, enabling machines to create art, mimic voices, and even generate entire worlds. This article delves deep into GANs, exploring their inception, inner workings, diverse applications, and the ethical considerations they raise.
Artificial Intelligence is a field that continues to evolve at a rapid pace, constantly pushing the boundaries of what machines can do. It has long been associated with problem-solving, data analysis, and automation, but the emergence of Generative Adversarial Networks (GANs) has unlocked a new dimension of AI’s capabilities: creativity. GANs have the power to generate realistic content, be it images, music, or even text, that is indistinguishable from human-created content. This revolutionary concept is altering how we approach AI and its applications across various domains.
In this article, we will embark on a comprehensive journey through the world of GANs. We will start with an introduction to GANs, delve into their history, explore their inner workings, and then investigate their wide-ranging applications. We will also address the ethical concerns that GANs have raised and offer insights into what the future holds for this groundbreaking technology.
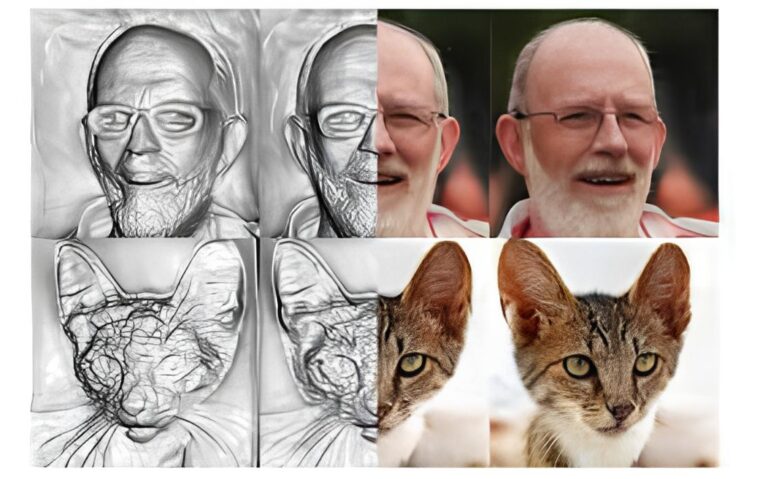
2. What are Generative Adversarial Networks (GANs)?
Generative Adversarial Networks, or GANs, represent a class of artificial intelligence algorithms designed to generate content that mimics human-created data. Unlike traditional AI systems that rely on explicit instructions and data input, GANs are trained to produce content by learning from existing data.
The core idea behind GANs is the existence of two neural networks, the generator and the discriminator, that engage in a competitive, adversarial process. The generator’s role is to create data that is as convincing as possible, while the discriminator’s task is to distinguish between real and generated data. This interplay of creation and judgment is what makes GANs unique and powerful.
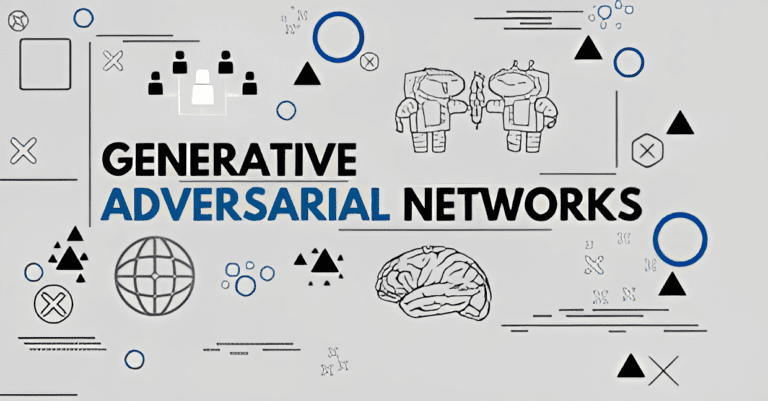
3. The Birth and Evolution of GANs
The concept of GANs was introduced by Ian Goodfellow and his colleagues in a seminal paper titled “Generative Adversarial Networks” published in 2014. Goodfellow’s idea was inspired by the human creative process, which often involves a tension between creation and critique.
The original GAN architecture consisted of a generator network and a discriminator network. The generator network would create data, while the discriminator network would evaluate it. Over time, the generator improved by learning from the discriminator’s feedback. The discriminator, in turn, improved its ability to distinguish real data from generated data. This adversarial process led to the refinement of both networks, resulting in highly realistic generated data.
Since the inception of GANs, the technology has seen rapid development. Researchers have proposed various GAN architectures and techniques to improve stability, training efficiency, and the quality of generated content. Some notable advancements include deep convolutional GANs (DCGANs), conditional GANs (cGANs), and progressive GANs (PGANs), among others.
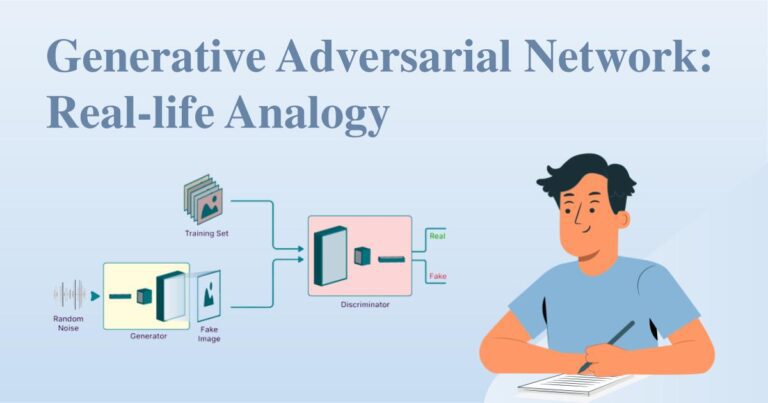
4. How Do GANs Work?
At the heart of GANs is a game-like framework involving two neural networks – the generator and the discriminator – that work in tandem to create and evaluate content. Let’s delve deeper into how this process works:
The Generator: The generator network starts with random noise as input and creates data. For example, in the case of generating images, the generator produces pixel data. Initially, the generated data is likely to be of poor quality and far from resembling the real data.
The Discriminator: The discriminator network is responsible for evaluating data, determining whether it is real or fake. It takes both real and generated data as input and assigns a probability that a given data point is real.
Training Process: During training, the generator and discriminator networks engage in a competitive process. The generator strives to create data that the discriminator cannot distinguish from real data, while the discriminator tries to improve its accuracy in distinguishing between real and generated data.
As training progresses, the generator gets better at creating realistic data, and the discriminator becomes more adept at differentiating between real and generated data. This competitive dynamic results in a generator capable of producing high-quality data that is often indistinguishable from human-created content.
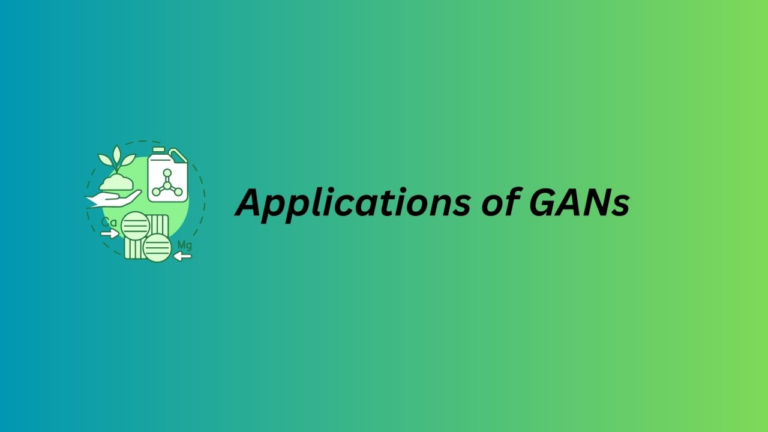
5. Applications of GANs
The applications of GANs are incredibly diverse and continue to expand. They have made a significant impact across multiple domains. Here are some notable applications:
5.1 Image Generation
GANs are widely recognized for their ability to generate realistic images. They have been employed in creating art, generating photorealistic scenes for video games, and even deepfake technology, which raises both creative and ethical concerns.
5.2 Style Transfer
GANs have been used to transfer the artistic style of one image to another, resulting in visually stunning effects. This has applications in design, photography, and the creative arts.
5.3 Super-Resolution
In image processing, GANs can be used to enhance the resolution of images, making them sharper and more detailed. This is beneficial in fields like medical imaging and satellite photography.
5.4 Face Aging and Rejuvenation
GANs can simulate how a person’s face might age over time or reverse the aging process. This has applications in entertainment, cosmetics, and law enforcement.
5.5 Text-to-Image Synthesis
GANs have the ability to generate images from textual descriptions, which can be valuable in fields like advertising and e-commerce.
5.6 Music and Audio Generation
GANs can be used to create music and audio that mimics different styles and artists. This has implications for the music industry and entertainment.
5.7 Deep Dream
Deep Dream is a technique that uses GANs to create surreal and hallucinogenic images. This is more of an artistic application and has gained popularity in the art world.
5.8 Data Augmentation
In machine learning, GANs are used to augment training data. They can generate additional data that is similar to the real dataset, improving model performance.
5.9 Drug Discovery
GANs have shown promise in generating molecular structures for drug development. They can help pharmaceutical companies identify potential drug candidates more efficiently.
5.10 Anomaly Detection
In cybersecurity, GANs can be used to detect anomalies in network traffic or user behavior, aiding in the identification of security threats.
These are just a few examples of how GANs are applied in various industries. Their versatility and creative potential make them a powerful tool for innovation.
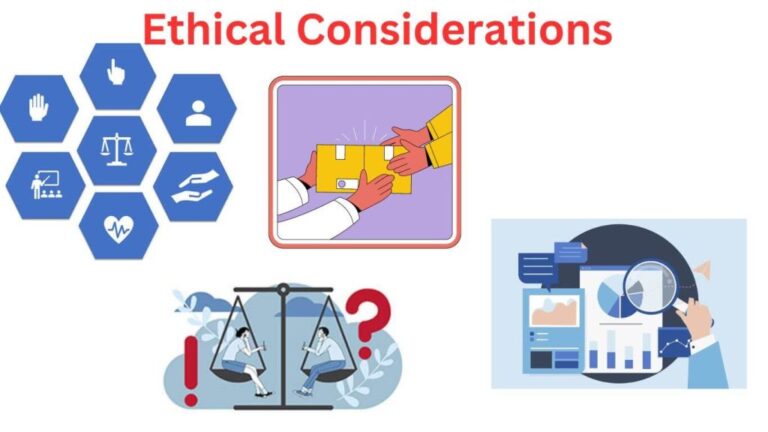
6. Ethical Considerations and Challenges
The rise of GANs has brought about a host of ethical concerns and challenges that need to be addressed:
6.1 Deepfakes and Misinformation
One of the most pressing issues is the use of GANs to create deepfake content, where individuals’ voices and images can be manipulated to create convincing fake videos. This technology has the potential for malicious use, including spreading misinformation, impersonation, and fraud.
6.2 Copyright and Intellectual Property
As GANs generate content that can closely resemble human-created works, issues surrounding copyright infringement and intellectual property rights have emerged. Determining the origin and ownership of generated content can be challenging.
6.3 Bias and Fairness
GANs can inherit biases present in the training data, leading to unfair or discriminatory outcomes. This is a significant concern, especially in applications like hiring algorithms and criminal justice systems.
6.4 Privacy
GANs can be used to create highly realistic fake images, which poses privacy risks. People may be depicted in compromising or inappropriate situations without their consent.
6.5 Accountability
Determining responsibility for the misuse of GANs can be challenging. Is it the responsibility of the developer, the user, or the platform hosting the technology?
Addressing these ethical concerns is crucial as GANs become more integrated into our daily lives. Striking a balance between creative freedom and responsible use is an ongoing challenge.
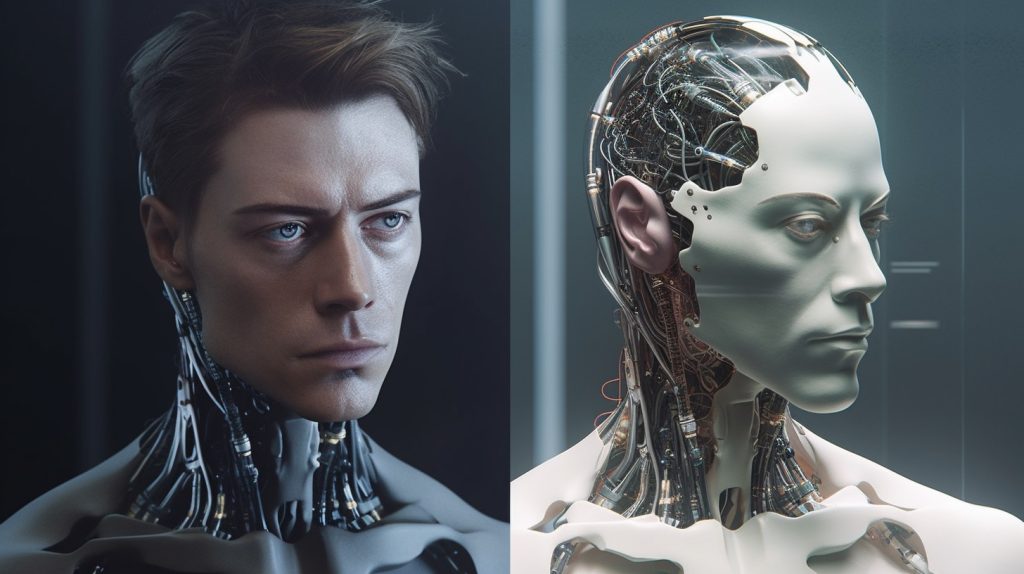
7. The Future of GANs
The future of Generative Adversarial Networks holds great promise. As the technology continues to evolve, we can anticipate several developments:
7.1 Enhanced Realism
GANs will produce content that is increasingly difficult to distinguish from human-created works. This will impact a wide range of creative industries, from entertainment to advertising.
7.2 Improved Ethical Guidelines
The ethical considerations surrounding GANs will lead to the development of stricter guidelines and regulations to ensure responsible use and prevent misuse.
7.3 Customization and Personalization
GANs will enable personalized content creation, from tailor-made products to individualized artistic expressions.
7.4 Industry-Specific Applications
GANs will be tailored to specific industries, resulting in highly specialized and powerful applications.
7.5 Democratization of Creativity
The availability of GAN tools and resources will democratize creativity, allowing more people to engage in content creation and artistry.
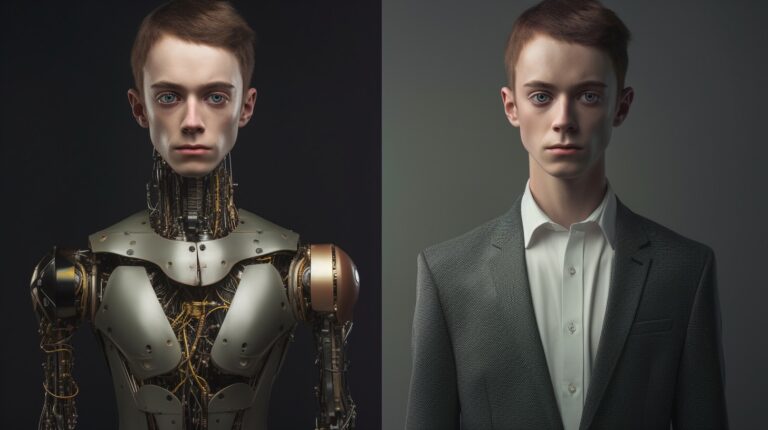
8. Conclusion
Generative Adversarial Networks represent a remarkable breakthrough in the field of artificial intelligence. They have pushed the boundaries of what machines can create and have had a transformative impact on numerous industries. However, this creative power also comes with ethical responsibilities and challenges that require careful consideration.
As GAN technology continues to advance, it will be essential to strike a balance between creative freedom and responsible use. The future of GANs promises even greater realism and personalization, making them an invaluable tool in the creative and innovative landscape of the future.
In closing, Generative Adversarial Networks are not just a technological innovation but a paradigm shift in how we harness the creative potential of AI. They are a testament to human ingenuity, opening doors to new possibilities and reshaping the way we view artificial intelligence. It’s an exciting journey that is only just beginning.