Introduction
Healthcare AI holds tremendous potential to revolutionize medical diagnostics, personalized treatment plans, and patient care. At the heart of these advancements lies the need for high-quality annotated data. Data annotation companies specializing in healthcare AI play a pivotal role in labeling medical images, clinical text, genomic data, and more, ensuring that AI algorithms can learn and make accurate predictions.
In this blog, we will explore the importance of data annotation in healthcare AI, discuss the types of annotation methods used, highlight key criteria for selecting annotation providers, and compare leading companies in the industry. We will then focus on SO Development, examining why they excel in delivering annotated data solutions tailored for healthcare AI applications.
Importance of Data Annotation in Healthcare AI
Data annotation is the process of labeling raw data to make it understandable for AI algorithms. In healthcare AI, annotated data is essential for training machine learning models that can assist healthcare professionals in various tasks:
- Medical Imaging: Annotated images enable AI algorithms to identify and analyze anomalies, tumors, and other medical conditions in radiology and pathology.
- Clinical Text and EHRs: Annotated clinical notes and electronic health records (EHRs) facilitate AI-driven insights into patient histories, treatment outcomes, and predictive analytics.
- Genomic Data: Annotated genomic data helps in identifying genetic variations, biomarkers, and personalized treatment options.
The accuracy and quality of annotations directly impact the performance and reliability of AI models in healthcare, making data annotation a critical step in the development and deployment of healthcare AI solutions.
Types of Data Annotation Methods in Healthcare AI
Data annotation methods vary based on the type of data and the specific AI application. Here are some common annotation techniques used in healthcare AI:
1. Image Annotation
- Bounding Boxes: Outline specific regions of interest in medical images, such as tumors or organs.
- Semantic Segmentation: Label each pixel in an image to distinguish different tissues or structures for detailed analysis.
- Landmark Annotation: Mark specific anatomical landmarks within medical images to assist in surgical planning and measurements.
2. Text Annotation
- Entity Recognition: Identify and classify entities in clinical text, such as patient demographics, medical conditions, or treatments.
- Medical Terminology Tagging: Label medical terms and concepts to enhance natural language processing (NLP) models for clinical documentation and information retrieval.
3. Time-Series Data Annotation
- Event Annotation: Label events or changes in physiological data (e.g., ECG waveforms or vital signs) to monitor patient health and detect anomalies.
4. Genomic Data Annotation
- Variant Annotation: Identify and classify genetic variants within genomic data to support research in personalized medicine and disease risk assessment.
Key Criteria for Selecting Data Annotation Providers in Healthcare AI
Choosing the right data annotation provider is crucial for healthcare organizations and AI developers aiming to leverage annotated data for medical applications. Consider the following criteria when evaluating potential providers:
1. Accuracy and Quality Control
Ensure that the annotation provider maintains rigorous quality control measures to deliver accurate and reliable annotations. Look for providers with expertise in healthcare AI and specialized knowledge of medical terminology and imaging standards.
2. Compliance with Regulatory Standards
Healthcare data is subject to stringent regulatory requirements, such as HIPAA in the United States and GDPR in Europe. Choose an annotation provider that adheres to regulatory standards and implements robust data security and confidentiality measures.
3. Domain Expertise and Experience
Select a provider with proven experience in healthcare AI and medical data annotation. Look for expertise in annotating diverse medical data types, familiarity with clinical workflows, and understanding of regulatory constraints.
4. Scalability and Flexibility
Evaluate the provider’s capacity to scale annotation services to accommodate large volumes of healthcare data while maintaining high quality and turnaround times. Flexible annotation solutions tailored to specific project requirements ensure seamless integration with AI development workflows.
5. Technology and Infrastructure
Assess the provider’s annotation tools, AI capabilities, and infrastructure to ensure compatibility with your AI development platform and data management systems. Advanced technologies, such as AI-assisted annotation tools and secure cloud-based platforms, can enhance efficiency and accuracy in data annotation processes.
6. Cost-Effectiveness and Transparency
Consider the provider’s pricing structure, including cost per annotation unit, volume discounts, and additional fees for specialized services. Transparent pricing and detailed cost estimates help manage budget constraints while ensuring quality and value in data annotation services.
7. Collaboration and Support
Effective communication and collaboration are essential for successful partnerships with data annotation providers. Choose a provider that offers responsive customer support, clear communication channels, and proactive project management to address any concerns or modifications promptly.
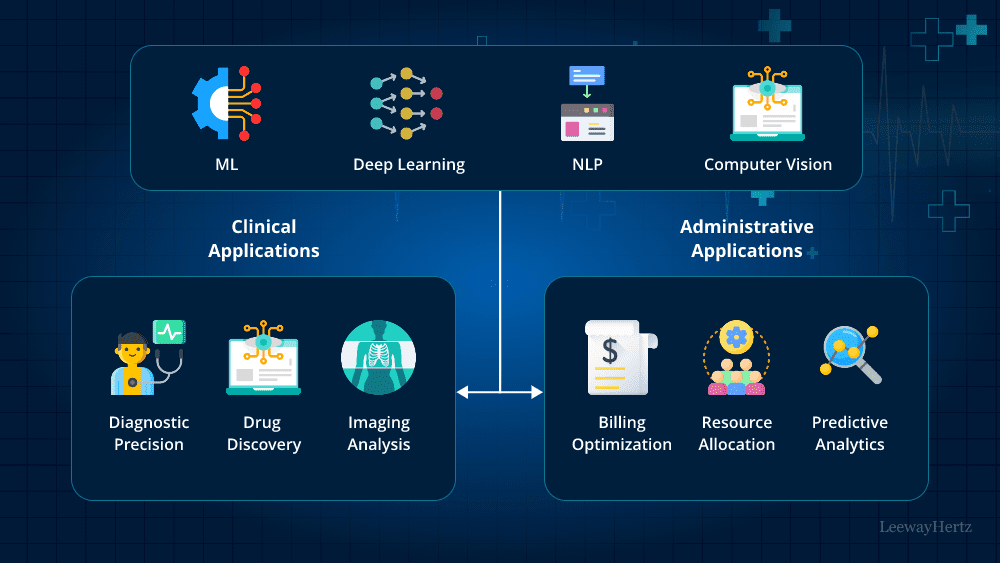
Best Practices for Selecting a Data Annotation Company
Define Your Requirements
Clearly outline your project’s requirements, including the type of data annotation needed, volume of data, accuracy expectations, and timeline. This will help you communicate effectively with potential providers and evaluate their capabilities.
Conduct Thorough Research
Research potential data annotation companies, considering factors such as their expertise, experience, and reputation in the industry. Review case studies, client testimonials, and any available performance metrics.
Request Samples and Demos
Request sample annotations or demos to assess the quality of work provided by the company. This will give you a firsthand look at their annotation accuracy, attention to detail, and overall quality.
Evaluate Technological Capabilities
Inquire about the tools and technologies used by the company. Advanced annotation tools and AI-assisted processes can significantly enhance efficiency and accuracy.
Assess Quality Control Measures
Quality control is critical to ensuring high-quality annotations. Inquire about the company’s quality assurance processes, including multi-layered reviews, automated checks, and regular audits.
Consider Scalability and Flexibility
Ensure the company can scale their services to meet your project’s needs and accommodate any changes in requirements. Flexibility in annotation workflows is also important for customization.
Verify Compliance and Security
Check that the company adheres to relevant data protection regulations and has robust data security measures in place. This is especially important when dealing with sensitive data.
Compare Pricing Models
Evaluate pricing models and structures to ensure they align with your budget. Look for transparent pricing without hidden fees and consider the overall value provided by the company.
Prioritize Customer Support and Collaboration
Effective communication and collaboration are essential for successful data annotation projects. Ensure the company provides regular updates, responsive support, and open channels for feedback.
Leading Data Annotation Companies for Healthcare AI
Let’s explore some of the leading data annotation companies specializing in healthcare AI and their unique strengths in delivering annotated data solutions for medical applications:
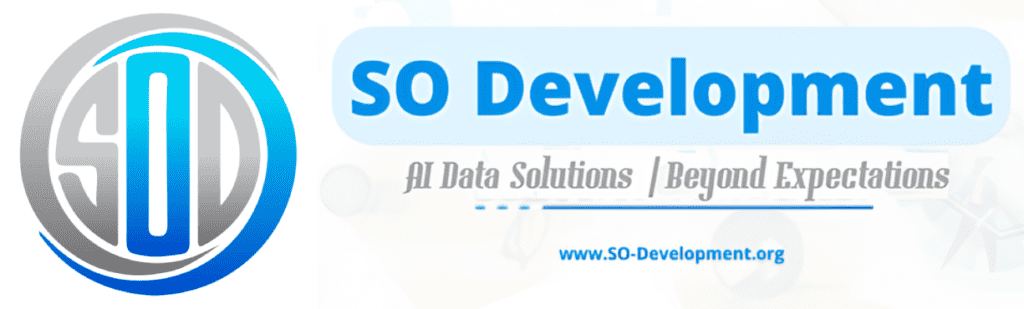
SO Development:
SO Development is a rising star in the data annotation landscape, with a specific focus on healthcare AI. Known for its innovative approach and commitment to quality, SO Development is making significant strides in the healthcare sector.
Services and Solutions
SO Development offers a comprehensive suite of annotation services tailored for healthcare:
- Medical Imaging Annotation: Annotating various medical images, including radiology, pathology, and ophthalmology images, to train AI models for accurate diagnosis and treatment planning.
- Text Annotation: Extracting valuable insights from clinical notes, EHRs, and other textual data to support AI-driven analysis and decision-making.
- Audio and Video Annotation: Labeling patient interviews, surgical videos, and other audio-visual data to develop AI systems for patient care and robotic surgery.
Technology and Expertise
SO Development combines cutting-edge technology with a team of skilled annotators, including healthcare professionals and data scientists. Their platform integrates seamlessly with various AI frameworks, making it easy for healthcare organizations to incorporate annotated data into their AI models. SO Development’s commitment to quality and innovation sets it apart in the healthcare AI landscape.
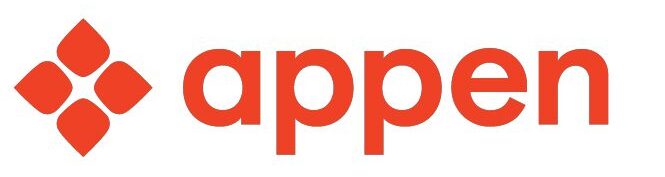
Appen
Appen is a global leader in data annotation, known for its extensive experience and expertise across various industries, including healthcare. Founded in 1996, Appen has a long track record of delivering high-quality annotation services.
Services and Solutions
Appen provides a comprehensive suite of annotation services for healthcare:
- Medical Imaging: Annotating radiology images, pathology slides, and other medical images to train AI diagnostic tools.
- Text Annotation: Labeling clinical notes, EHRs, and other textual data to support NLP applications in healthcare.
- Audio Annotation: Labeling patient interviews and voice recordings for developing AI systems that can understand and respond to spoken language.
Technology and Expertise
Appen leverages a combination of human expertise and machine-learning techniques to deliver high-quality annotations. Their global network of annotators includes healthcare professionals, ensuring that annotations are both accurate and clinically relevant.

Lionbridge AI
Lionbridge AI, a division of Lionbridge, is a global leader in data annotation with extensive experience in healthcare. Founded in 1996, Lionbridge has a long history of providing high-quality annotation services across various industries.
Services and Solutions
Lionbridge AI offers a comprehensive suite of annotation services for healthcare:
- Medical Imaging: Annotating radiology images, pathology slides, and other medical images to train AI diagnostic tools.
- Text Annotation: Labeling clinical notes, EHRs, and other textual data to support NLP applications in healthcare.
- Audio Annotation: Labeling patient interviews and voice recordings for developing AI systems that can understand and respond to spoken language.
Technology and Expertise
Lionbridge AI leverages a combination of human expertise and machine learning techniques to deliver high-quality annotations. Their global network of annotators includes healthcare professionals, ensuring that annotations are both accurate and clinically relevant.
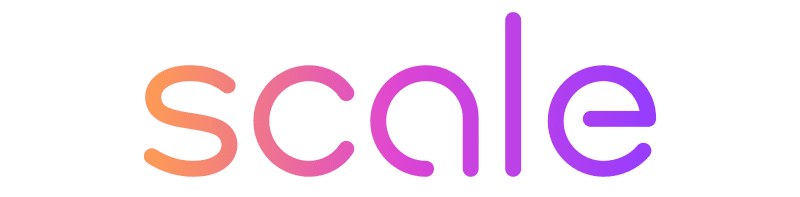
Scale AI
Scale AI is a leading player in the data annotation market, offering comprehensive solutions tailored for healthcare AI. Founded in 2016, Scale AI has quickly risen to prominence due to its robust platform and high-quality annotation services.
Services and Solutions
Scale AI provides a suite of services tailored for healthcare, including:
- Medical Image Annotation: Annotating radiology images, MRIs, CT scans, and X-rays to train diagnostic AI systems.
- Text Annotation: Labeling electronic health records (EHRs) and clinical notes to extract valuable insights and support natural language processing (NLP) applications.
- Video Annotation: Annotating surgical videos to assist in the development of robotic surgery systems and training AI models to recognize procedural steps.
Technology and Expertise
Scale AI leverages advanced machine learning techniques and a team of skilled annotators, including healthcare professionals, to ensure the highest quality annotations. Their platform integrates seamlessly with various AI frameworks, making it easy for healthcare organizations to incorporate annotated data into their AI models.
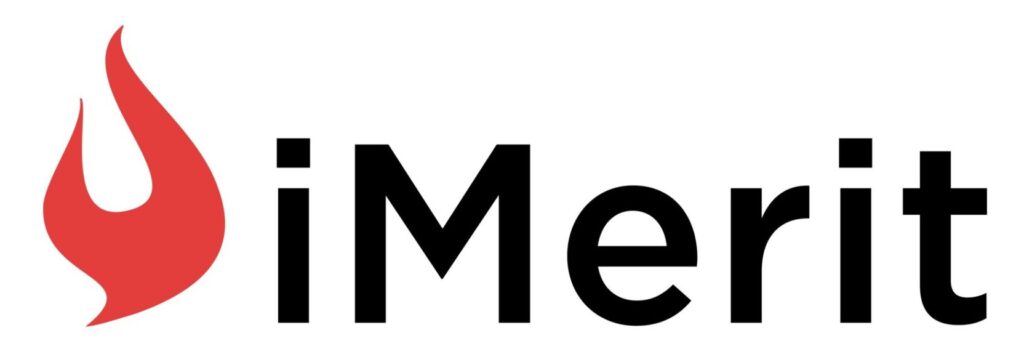
iMerit
iMerit is a leading data annotation company with a strong focus on healthcare. Founded in 2012, iMerit has built a reputation for delivering high-quality annotation services across various industries, with a significant emphasis on healthcare.
Services and Solutions
iMerit offers a wide range of annotation services for healthcare applications:
- Medical Imaging: Annotating radiology images, pathology slides, and other medical images to train AI diagnostic tools.
- Text Annotation: Labeling clinical notes, EHRs, and other textual data to support NLP applications in healthcare.
- Audio and Video Annotation: Annotating surgical videos and patient interviews to develop advanced AI systems for healthcare.
Technology and Expertise
iMerit combines human expertise with advanced technology to deliver precise annotations. Their team includes medical professionals and data scientists who ensure that annotations are accurate and clinically relevant.
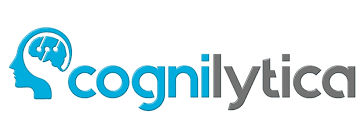
Cognilytica
Cognilytica is a market research and advisory firm that also provides data annotation services, focusing on healthcare AI. With a deep understanding of the AI landscape, Cognilytica offers specialized annotation services to meet the unique needs of healthcare organizations.
Services and Solutions
Cognilytica’s healthcare-focused services include:
- Medical Imaging: Annotating radiology and pathology images to train diagnostic AI models.
- Clinical Data: Labeling EHRs and clinical notes to extract structured information for AI analysis.
- Genomic Data: Annotating genetic sequences to support AI-driven research in genomics and personalized medicine.
Technology and Expertise
Cognilytica leverages its extensive market research expertise to provide high-quality annotation services. Their team includes experts in healthcare and AI, ensuring that annotations meet the rigorous standards required in the medical field.
Conclusion
In conclusion, selecting the right data annotation provider is crucial for healthcare organizations and AI developers embarking on transformative AI initiatives. By prioritizing factors such as accuracy, compliance, scalability, innovation, pricing transparency, and customer support, healthcare stakeholders can make informed decisions that drive the success of healthcare AI projects.
SO Development emerges as a top choice among data annotation companies specializing in healthcare AI, thanks to its specialization in healthcare AI, commitment to quality and accuracy, scalability, compliance with regulatory standards, technological capabilities, transparent pricing models, and customer-centric approach. By partnering with SO Development, healthcare organizations can harness the power of accurately annotated data to accelerate medical innovation, improve patient care, and achieve sustainable healthcare outcomes in the era of AI-driven healthcare transformation.