Introduction
Conversational AI, an interdisciplinary field at the intersection of artificial intelligence, machine learning, and natural language processing, has witnessed remarkable advancements in recent years. These advancements have been driven by the pursuit of more human-like interactions between machines and humans. Among the myriad of challenges in this endeavor, recognizing and appropriately responding to human emotions stands out as a critical aspect. Emotion recognition in conversational AI systems holds immense potential to enhance user experience, enable more empathetic interactions, and facilitate deeper engagement. In this article, we delve into the significance of emotion recognition in conversational AI, exploring its underlying principles, current implementations, challenges, and future prospects.
Key Components of Emotion Recognition in Conversational AI
- Facial Expression Analysis: Facial expression analysis involves detecting and interpreting facial cues to infer emotional states. This can be achieved through computer vision techniques such as facial landmark detection, feature extraction, and classification algorithms.
- Speech Emotion Recognition: Speech carries valuable emotional information through variations in pitch, tone, intensity, and speech patterns. Speech emotion recognition algorithms analyze these acoustic features to identify underlying emotional states accurately.
- Textual Emotion Analysis: Textual content in conversations provides insights into the emotional state of the participants. Natural language processing (NLP) techniques, including sentiment analysis and emotion detection, are employed to extract emotional cues from textual data.
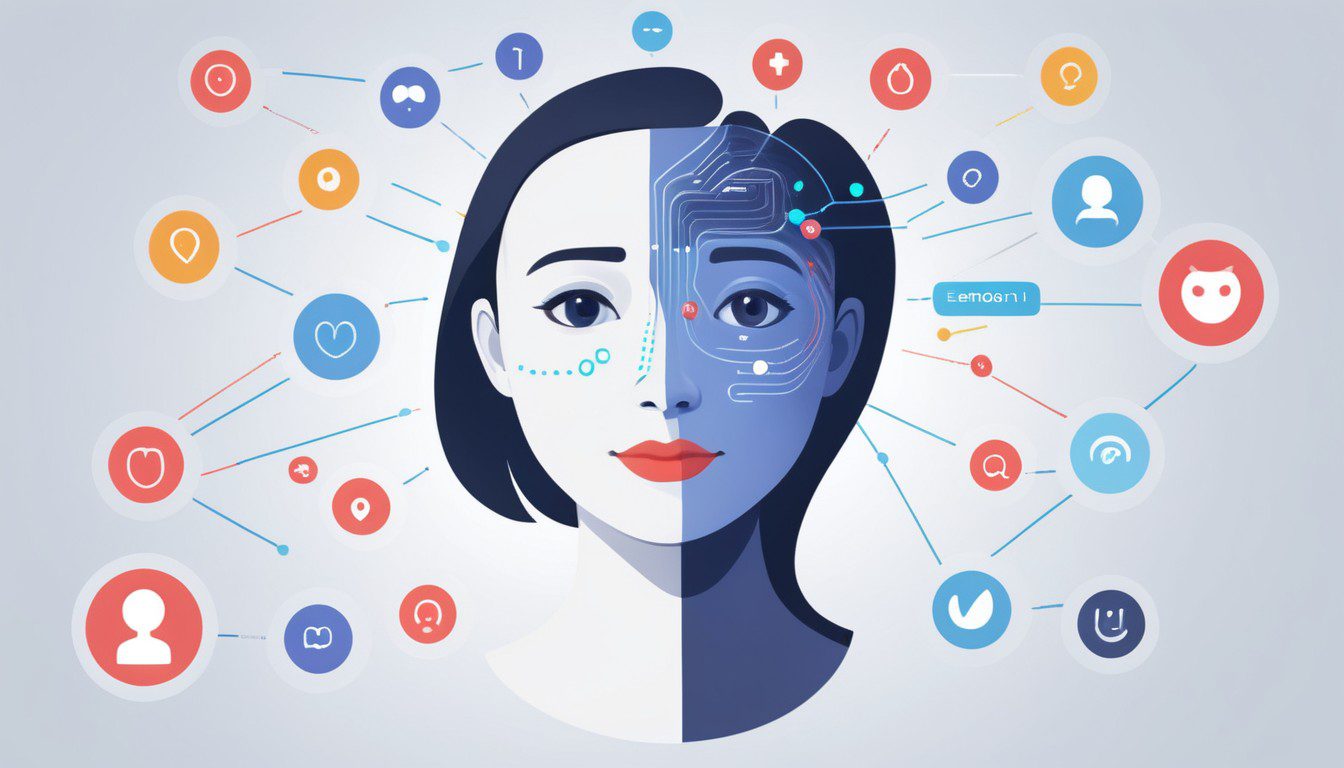
Current Implementations and Applications
Emotion recognition technologies have found widespread applications across various domains, including customer service, healthcare, education, and entertainment.
- Customer Service: Chatbots and virtual assistants equipped with emotion recognition capabilities can offer personalized and empathetic support to users. By analyzing user sentiment in real-time, these systems can adapt their responses to better address user needs and concerns.
- Mental Health Support: Emotion-aware conversational agents hold promise in providing mental health support and counseling services. These systems can detect signs of distress or emotional instability in users’ speech or text and offer appropriate interventions or referrals to professional help.
- Education and Training: Emotion-aware educational platforms can enhance learning experiences by adapting content delivery based on students’ emotional states. By gauging students’ engagement and frustration levels, these systems can provide tailored feedback and support to optimize learning outcomes.
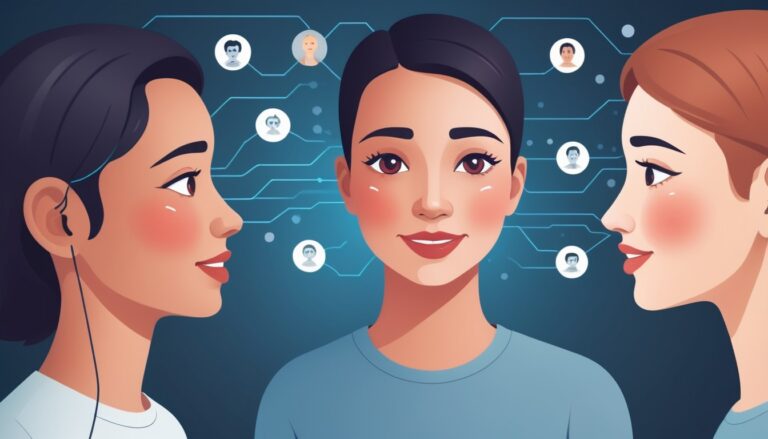
Challenges and Limitations
- Despite the advancements in emotion recognition technologies, several challenges and limitations persist:
- Ambiguity and Context: Emotions are complex and often influenced by contextual factors, making accurate recognition challenging, especially in ambiguous situations.
- Cross-cultural Variability: Emotion expression and interpretation vary across cultures, posing challenges for universal emotion recognition models.
- Privacy and Ethical Concerns: Emotion recognition technologies raise concerns regarding privacy intrusion and ethical implications, particularly in sensitive domains such as healthcare and surveillance.
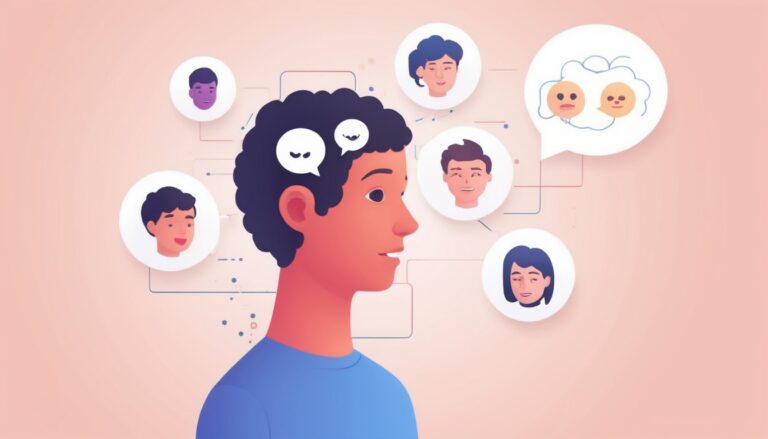
Future Prospects and Emerging Trends
The future of emotion recognition in conversational AI holds exciting possibilities, driven by advancements in AI, deep learning, and affective computing:
- Multimodal Fusion: Integrating multiple modalities such as facial expressions, speech, and textual content can improve the robustness and accuracy of emotion recognition systems.
- Contextual Understanding: Emotion recognition models will evolve to incorporate contextual understanding, leveraging contextual cues to infer more nuanced emotional states accurately.
- Ethical AI Design: Emphasis will be placed on developing ethically responsible emotion recognition systems that prioritize user privacy, transparency, and fairness.
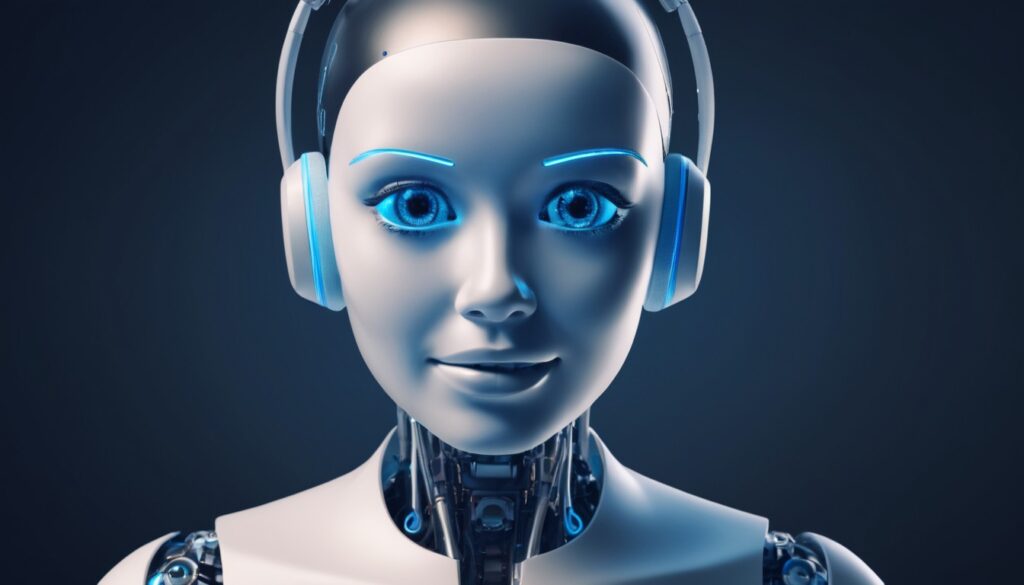
Conclusion
Emotion recognition plays a pivotal role in advancing the capabilities of conversational AI systems, enabling more empathetic, personalized, and engaging interactions. As technology continues to evolve, addressing the challenges and ethical considerations associated with emotion recognition will be crucial in harnessing its full potential for the benefit of society. Embracing a human-centered approach, grounded in empathy and understanding, will pave the way for the next generation of emotionally intelligent conversational AI systems.