Introduction
Artificial Intelligence (AI) depends fundamentally on the quality and quantity of training data. Without sufficient, diverse, and accurate datasets, even the most sophisticated algorithms underperform or behave unpredictably. Traditional data collection methods — surveys, expert labeling, in-house data curation — can be expensive, slow, and limited in scope. Crowdsourcing emerged as a powerful alternative: leveraging distributed human labor to annotate, generate, validate, or classify data efficiently and at scale.
However, crowdsourcing also brings major ethical, operational, and technical challenges that, if ignored, can undermine AI systems’ fairness, transparency, and robustness. Especially as AI systems move into sensitive areas such as healthcare, finance, and criminal justice, ensuring responsible crowdsourced data practices is no longer optional — it is essential.
This guide provides a deep, comprehensive overview of the ethical principles, major obstacles, and best practices for successfully and responsibly scaling crowdsourced AI training data collection efforts.
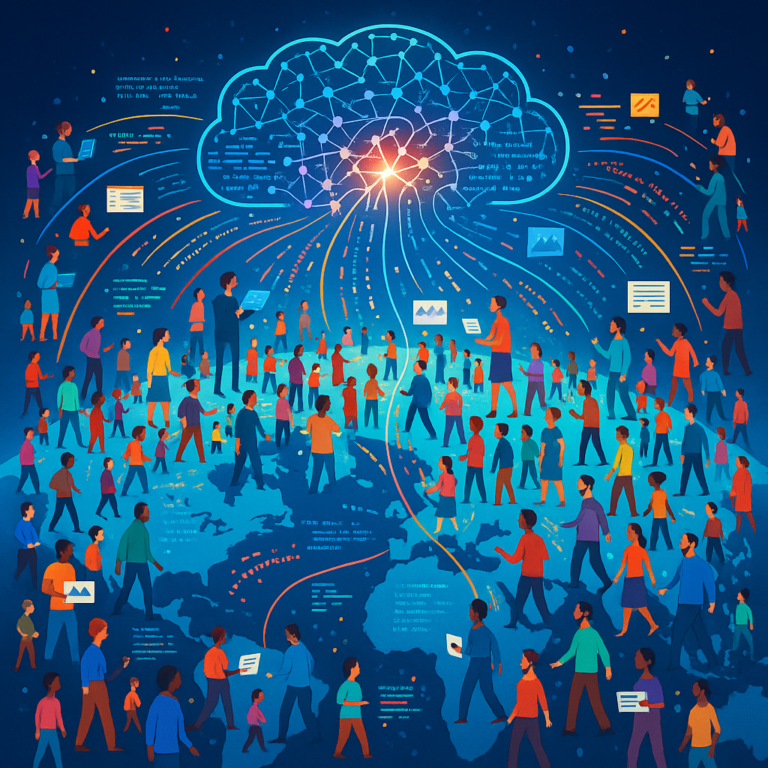
Understanding Crowdsourced AI Training Data
What is Crowdsourcing in AI?
Crowdsourcing involves outsourcing tasks traditionally performed by specific agents (like employees or contractors) to a large, undefined group of people via open calls or online platforms. In AI, tasks could range from simple image tagging to complex linguistic analysis or subjective content judgments.
Core Characteristics of Crowdsourced Data:
Scale: Thousands to millions of data points created quickly.
Diversity: Access to a wide array of backgrounds, languages, perspectives.
Flexibility: Rapid iteration of data collection and adaptation to project needs.
Cost-efficiency: Lower operational costs compared to hiring full-time annotation teams.
Real-time feedback loops: Instant quality checks and corrections.
Types of Tasks Crowdsourced:
Data Annotation: Labeling images, text, audio, or videos with metadata for supervised learning.
Data Generation: Creating new examples, such as paraphrased sentences, synthetic dialogues, or prompts.
Data Validation: Reviewing and verifying pre-existing datasets to ensure accuracy.
Subjective Judgment Tasks: Opinion-based labeling, such as rating toxicity, sentiment, emotional tone, or controversy.
Content Moderation: Identifying inappropriate or harmful content to maintain dataset safety.
Examples of Applications:
Annotating medical scans for diagnostic AI.
Curating translation corpora for low-resource languages.
Building datasets for content moderation systems.
Training conversational agents with human-like dialogue flows.
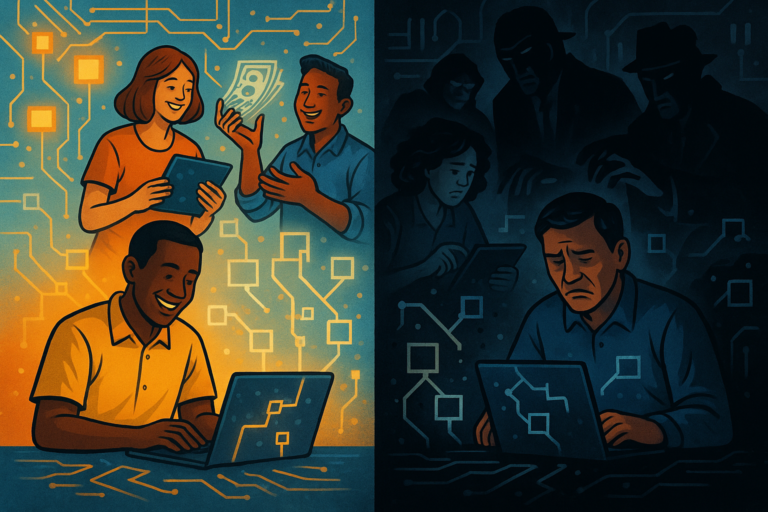
The Ethics of Crowdsourcing AI Data
Fair Compensation
Low compensation has long plagued crowdsourcing platforms. Studies show many workers earn less than local minimum wages, especially on platforms like Amazon Mechanical Turk (MTurk). This practice is exploitative, erodes worker trust, and undermines ethical AI.
Best Practices:
Calculate estimated task time and offer at least minimum wage-equivalent rates.
Provide bonuses for high-quality or high-volume contributors.
Publicly disclose payment rates and incentive structures.
Informed Consent
Crowd workers must know what they’re participating in, how the data they produce will be used, and any potential risks to themselves.
Best Practices:
Use clear language — avoid legal jargon.
State whether the work will be used in commercial products, research, military applications, etc.
Offer opt-out opportunities if project goals change significantly.
Data Privacy and Anonymity
Even non-PII data can become sensitive when aggregated or when AI systems infer unintended attributes (e.g., health status, political views).
Best Practices:
Anonymize contributions unless workers explicitly consent otherwise.
Use encryption during data transmission and storage.
Comply with local and international data protection regulations.
Bias and Representation
Homogenous contributor pools can inject systemic biases into AI models. For example, emotion recognition datasets heavily weighted toward Western cultures may misinterpret non-Western facial expressions.
Best Practices:
Recruit workers from diverse demographic backgrounds.
Monitor datasets for demographic skews and correct imbalances.
Apply bias mitigation algorithms during data curation.
Transparency
Opacity in data sourcing undermines trust and opens organizations to criticism and legal challenges.
Best Practices:
Maintain detailed metadata: task versions, worker demographics (if permissible), time stamps, quality control history.
Consider releasing dataset datasheets, as proposed by leading AI ethics frameworks.
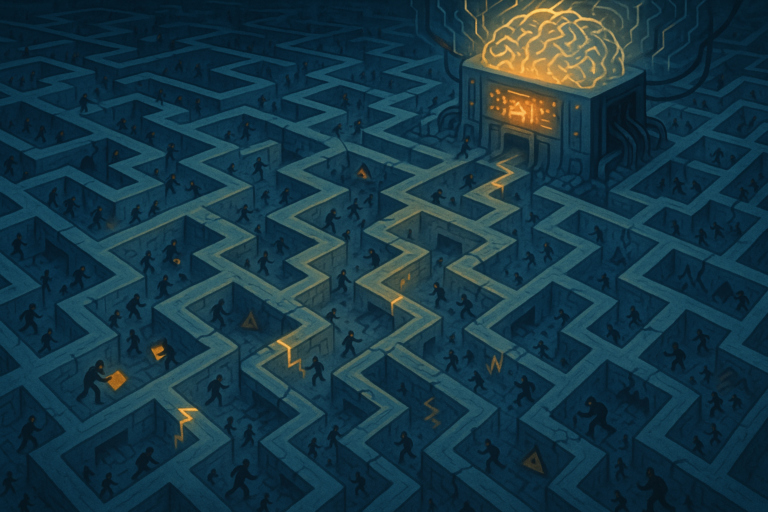
Challenges of Crowdsourced Data Collection
Ensuring Data Quality
Quality is variable in crowdsourcing because workers have different levels of expertise, attention, and motivation.
Solutions:
Redundancy: Have multiple workers perform the same task and aggregate results.
Gold Standards: Seed tasks with pre-validated answers to check worker performance.
Dynamic Quality Weighting: Assign more influence to consistently high-performing workers.
Combatting Fraud and Malicious Contributions
Some contributors use bots, random answering, or “click-farming” to maximize earnings with minimal effort.
Solutions:
Include trap questions or honeypots indistinguishable from normal tasks but with known answers.
Use anomaly detection to spot suspicious response patterns.
Create a reputation system to reward reliable contributors and exclude bad actors.
Task Design and Worker Fatigue
Poorly designed tasks lead to confusion, lower engagement, and sloppy work.
Solutions:
Pilot test all tasks with a small subset of workers before large-scale deployment.
Provide clear examples of good and bad responses.
Keep tasks short and modular (2-10 minutes).
Motivating and Retaining Contributors
Crowdsourcing platforms often experience high worker churn. Losing trained, high-performing workers increases costs and degrades quality.
Solutions:
Offer graduated bonus schemes for consistent contributors.
Acknowledge top performers in public leaderboards (while respecting anonymity).
Build communities through forums, feedback sessions, or even competitions.
Managing Scalability
Scaling crowdsourcing from hundreds to millions of tasks without breaking workflows requires robust systems.
Solutions:
Design modular pipelines where tasks can be easily divided among thousands of workers.
Automate the onboarding, qualification testing, and quality monitoring stages.
Use API-based integration with multiple crowdsourcing vendors to balance load.
Managing Emergent Ethical Risks
New, unexpected risks often arise once crowdsourcing moves beyond pilot stages.
Solutions:
Conduct regular independent ethics audits.
Set up escalation channels for workers to report concerns.
Update ethical guidelines dynamically based on new findings.
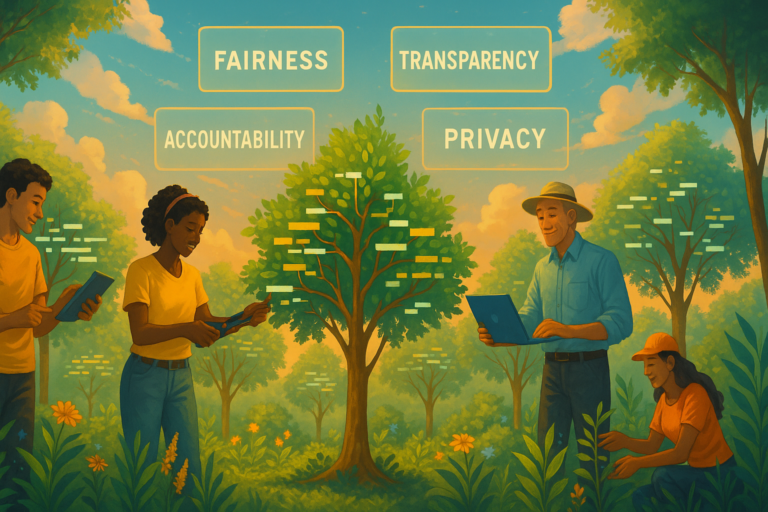
Best Practices for Scalable and Ethical Crowdsourcing
Area | Detailed Best Practices |
---|---|
Worker Management | – Pay living wages based on region-specific standards. – Offer real-time feedback during tasks. – Respect opt-outs without penalty. – Provide clear task instructions and sample outputs. – Recognize workers’ cognitive labor as valuable. |
Quality Assurance | – Build gold-standard examples into every task batch. – Randomly sample and manually audit a subset of submissions. – Introduce “peer review” where workers verify each other. – Use consensus mechanisms intelligently rather than simple majority voting. |
Diversity and Inclusion | – Recruit globally, not just from Western markets. – Track gender, race, language, and socioeconomic factors. – Offer tasks in multiple languages and dialects. – Regularly analyze demographic gaps and course-correct. |
Ethical Compliance | – Write plain-language consent forms. – Avoid tasks that could cause psychological harm. – Do not exploit vulnerable populations. – Publish ethical review findings annually. |
Technology and Tools | – Build dynamic workflow engines that adjust based on worker speed, quality, and engagement. – Use AI-assisted quality checking but retain human oversight. – Log all worker interactions and task metadata for future audits. |
Data Documentation | – Maintain “Datasheets for Datasets” (Gebru et al., 2018). – Document known biases, limitations, and collection conditions. – Release transparency reports where feasible. – Involve external reviewers to validate claims. |
Continuous Improvement | – Send post-task surveys to collect worker feedback. – Analyze error types and revise instructions iteratively. – Offer quarterly updates to workers about project progress. – Reward innovation in improving task execution methods. |
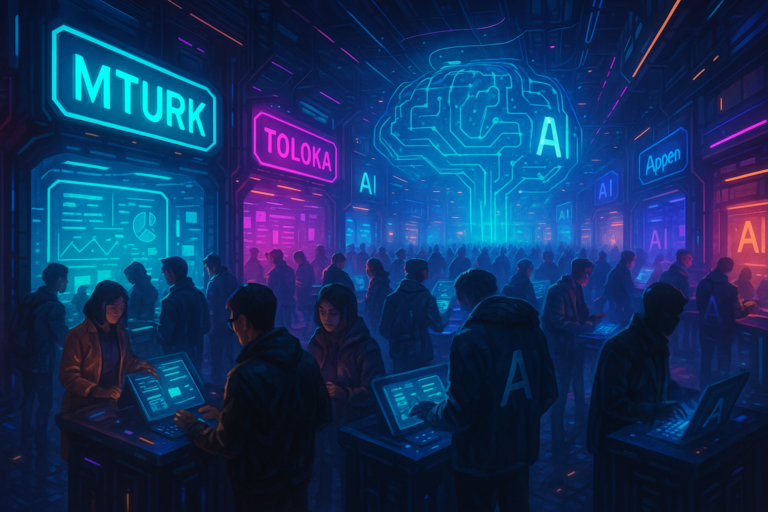
Platforms and Tools Commonly Used
Pros: High volume, mature platform.
Cons: Often criticized for low wages, worker exploitation, lack of protections.
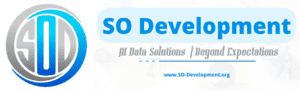
Appen and Lionbridge
Pros: Enterprise-grade workflows, quality assurance layers.
Cons: Costlier, slower to scale.
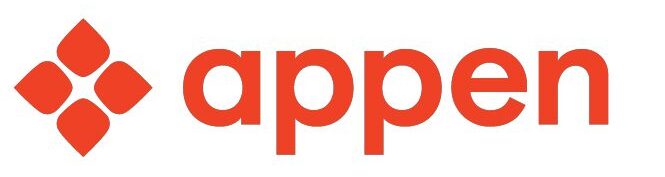
Pros: Strong multilingual capabilities, emerging leader for non-English tasks.
Cons: Limited documentation for English-only users.
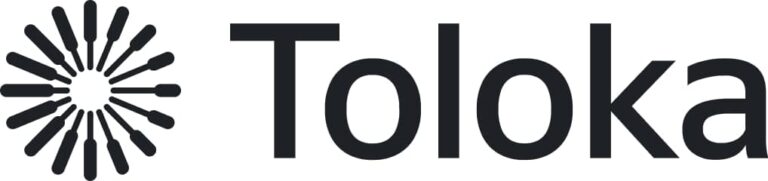
Pros: Focus on quality over quantity, better pay for workers.
Cons: Higher cost per label compared to bulk crowdsourcing.
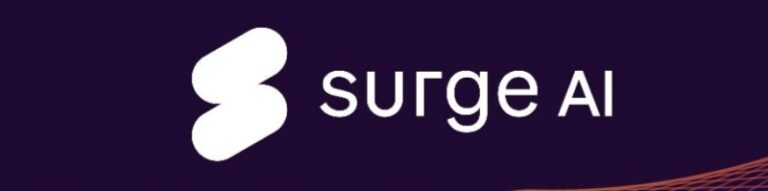
Pros: Offer hybrid AI-human solutions, great for complex data (e.g., medical imaging).
Cons: May not allow detailed control over worker engagement practices.
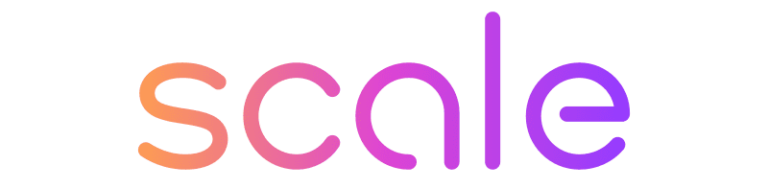
Real-World Examples
OpenAI Fine-tuning:
OpenAI relied on globally distributed contributors to build Reinforcement Learning from Human Feedback (RLHF) datasets. Workers received detailed training and were paid relatively well compared to industry standards.
Google Jigsaw’s Perspective API:
Developed multilingual toxicity detection tools by crowdsourcing labels in multiple languages. Faced challenges around defining “toxicity” consistently across cultures.
Meta’s No Language Left Behind (NLLB) Project:
Crowdsourced native speakers for over 200 languages, many low-resource, to train translation models. Used careful screening to avoid urban and elite-only samples.
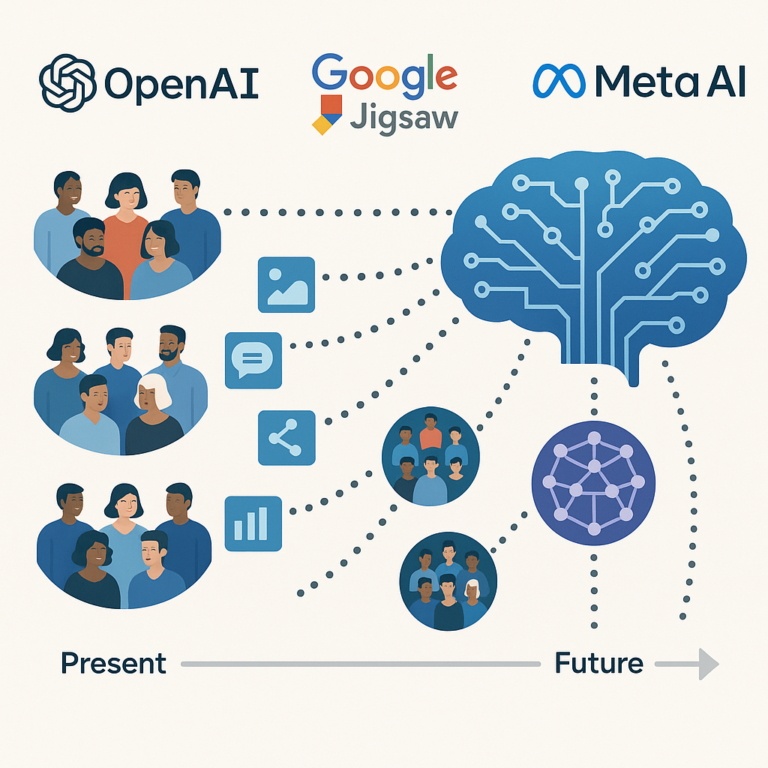
Future Directions in Crowdsourced AI Data
Federated Crowdsourcing
Workers collect and label data on-device with full privacy, transmitting only model updates (not raw data).
Active Learning Integration
Only uncertain or borderline examples are sent to workers, dramatically reducing labeling costs while maximizing impact.
Incentivized Ethical AI Programs
Workers are rewarded not just for labeling speed but for spotting biases, harms, or safety issues in early AI outputs.
Synthetic Data Crowdsourcing
Humans supervise and refine AI-generated synthetic data, guiding it toward high-quality, ethically robust training examples.
Community-driven AI Data Models
Future data collection may shift toward co-creation: AI communities (researchers + users) collaboratively building and owning datasets, creating more equitable AI futures.
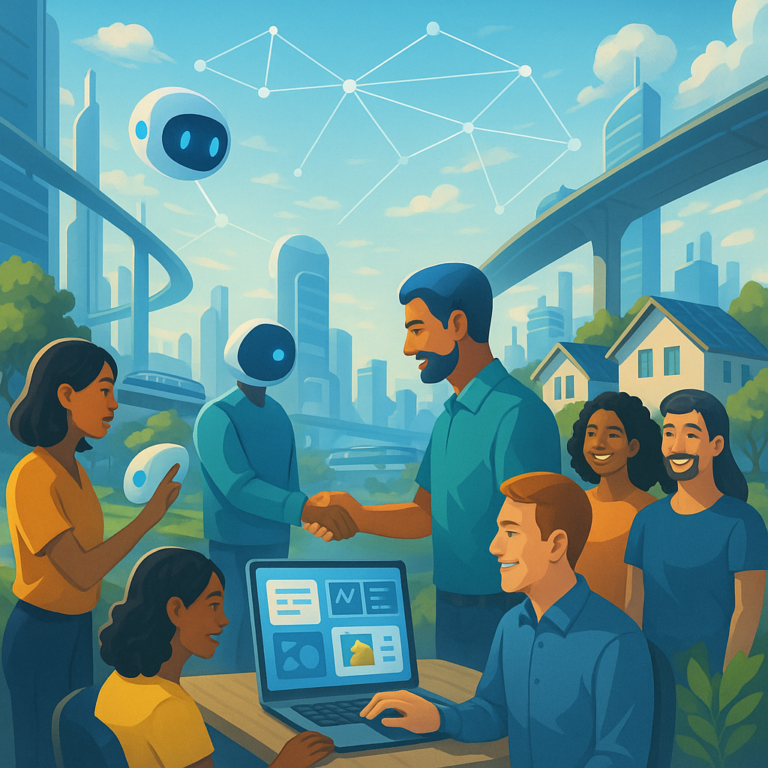
Conclusion
Crowdsourcing has transformed the data collection landscape for AI, enabling the creation of models that are smarter, faster, and more globally aware. Yet this power must be wielded with great care. Ethical failures at the data stage echo through to AI products, amplifying biases, injustices, and risks at scale. Organizations serious about building responsible AI must center worker welfare, data quality, privacy, and transparency at every stage of their crowdsourcing pipelines.
By embracing the principles and practices detailed here, AI practitioners can ensure their crowdsourced datasets are not merely large, but just, diverse, and powerful engines for positive innovation.