Introduction: The Foundation of AI Success
In the realm of artificial intelligence (AI) and machine learning (ML), data labeling is the cornerstone of success. A well-labeled dataset enables AI models to learn, predict, and perform tasks with accuracy and reliability. However, with the growing demand for labeled data, the market for labeling platforms has become vast and varied. Choosing the right platform is not just about convenience—it’s about achieving quality, scalability, and cost-efficiency.
This guide dives deep into the process of selecting the best labeling platform tailored to your needs, highlighting the leading platforms, evaluating critical features, and addressing challenges.
Understanding Your Data Labeling Needs
Before diving into platform features, you need to understand the nature and scope of your data.
1. Data Types to Label
Different platforms specialize in various data types.
- Images: Object detection, segmentation, classification (e.g., bounding boxes, polygons).
- Videos: Frame-by-frame annotation, object tracking.
- Text: Sentiment analysis, named entity recognition, text classification.
- Audio: Speaker identification, transcription, sound event detection.
- 3D Data (LiDAR/Point Clouds): Autonomous driving, spatial mapping.
2. Project Scope
- Small-scale Projects: Platforms like CVAT or LabelImg are great for compact datasets.
- Large-scale Enterprise Projects: Platforms like Scale AI or Labelbox provide scalable infrastructure.
3. Annotation Complexity
For intricate projects like multi-class segmentation or LiDAR point clouds, prioritize platforms with advanced tools and automation.
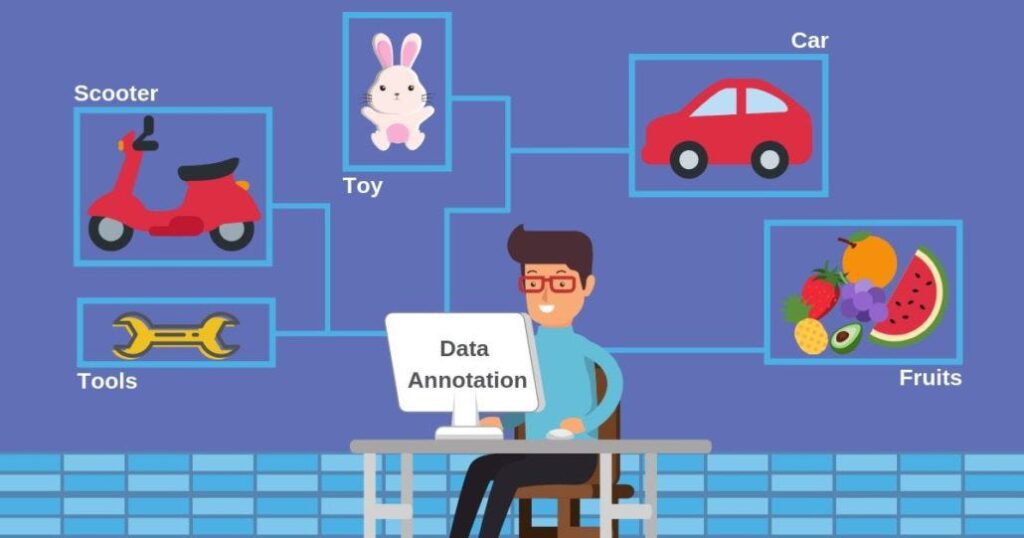
Key Factors to Evaluate
1. Ease of Use
A user-friendly platform increases productivity and reduces training time for annotators. Look for:
- Drag-and-drop features.
- Intuitive user interfaces.
- Training resources and tutorials.
2. Support for Automation
Platforms offering AI-assisted tools like pre-labeling, active learning, and auto-suggestions can save significant time and resources. Examples include:
- Labelbox: AI-powered labeling suggestions.
- Supervisely: Pre-trained models for initial annotation.
3. Collaboration Tools
For team projects, prioritize platforms with collaboration features like:
- Multi-user access.
- Real-time progress tracking.
- Built-in communication tools.
4. Quality Control Mechanisms
Annotation quality is critical. Look for:
- Consensus models (majority vote system).
- Review workflows with tiered approval.
- Built-in validation processes.
5. Integration with AI/ML Pipelines
Seamless integration with frameworks like TensorFlow, PyTorch, or cloud platforms such as AWS or Google Cloud accelerates workflows.
6. Pricing Models
Understand the cost structure—pay-per-annotation, subscription-based, or open-source platforms—and choose what aligns with your budget.
Top Labeling Platforms to Consider
Here’s a list of platforms excelling in 2024, along with their strengths:
SO Development
- Strengths: Advanced AI-driven tools, highly scalable enterprise solutions, and robust collaboration features designed for streamlined workflows.
- Use Case: Perfect for handling large datasets across diverse annotation types, including image, text, and video, with a focus on accuracy and efficiency.
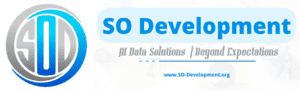
Labelbox
- Strengths: AI-driven tools, enterprise-grade scalability, excellent collaboration features.
- Use Case: Ideal for large datasets requiring multiple annotation types (image, text, video).
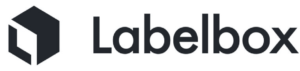
Supervisely
- Strengths: Advanced image and video annotation tools, customization, automation-friendly.
- Use Case: Preferred for AI-driven image recognition projects.
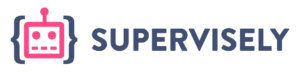
CVAT (Computer Vision Annotation Tool)
- Strengths: Open-source, flexible, and supports complex annotations like LiDAR.
- Use Case: Best for budget-conscious teams with technical expertise.
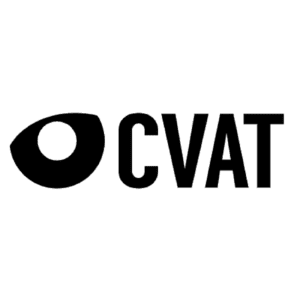
Scale AI
- Strengths: Enterprise-level performance, emphasis on quality control, robust AI automation.
- Use Case: Autonomous vehicle projects, massive-scale labeling.
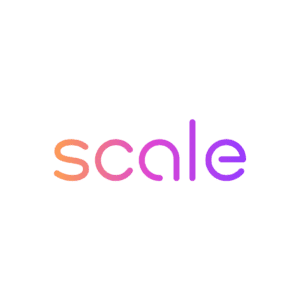
Amazon SageMaker Ground Truth
- Strengths: Tight integration with AWS, automated labeling options.
- Use Case: End-to-end AI model training pipelines.
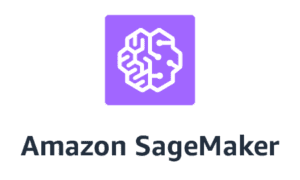
Challenges in Labeling and How Platforms Solve Them
1. Managing Annotation Accuracy
- Problem: Inconsistent annotations lead to poor model performance.
- Solution: Use platforms with consensus models or a review system, like Scale AI or Labelbox.
2. Handling Large-Scale Projects
- Problem: Datasets grow exponentially, leading to delays.
- Solution: Platforms with scalable infrastructure (e.g., Supervisely, Scale AI).
3. Budget Constraints
- Problem: High-quality platforms can be expensive.
- Solution: Opt for open-source solutions like CVAT or subscription plans that align with your usage.
4. Complex Data Types
- Problem: Lack of support for 3D or multi-modal data.
- Solution: Choose platforms like Supervisely or CVAT for LiDAR or point cloud data.
Future Trends in Data Labeling Platforms
1. Increased Automation
AI-driven annotation tools are reducing manual efforts and improving efficiency.
2. Multi-Modal Annotation
Platforms now support simultaneous labeling of image, text, and video datasets for unified AI models.
3. Focus on User Experience
Intuitive designs and low-code interfaces are becoming industry standards.
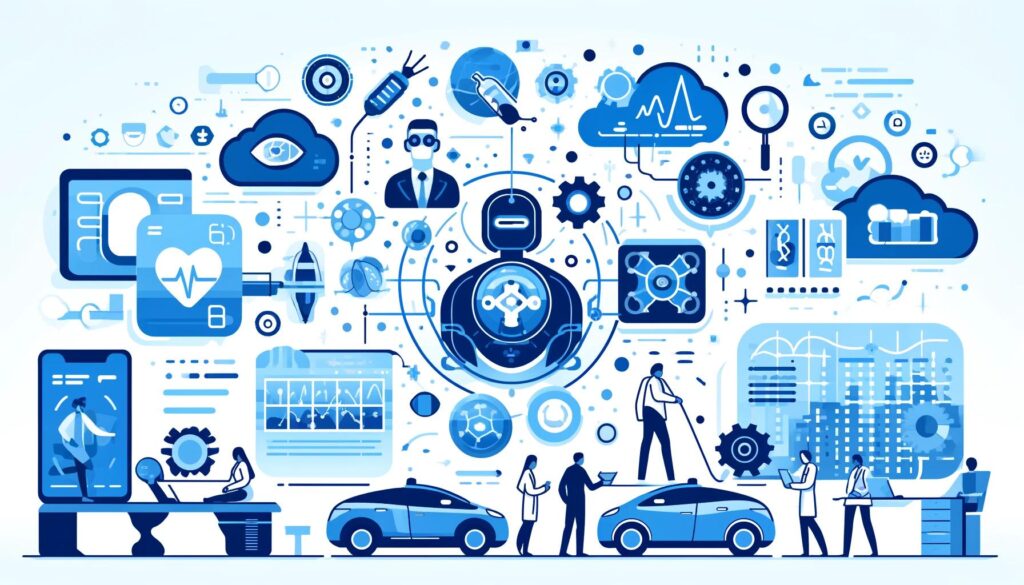
How to Make Your Final Choice
- Run a Pilot Project: Test multiple platforms on a small dataset to evaluate performance and usability.
- Analyze ROI: Compare the cost versus quality and time saved using each platform.
- Seek Recommendations: Consult industry peers or reviews for insights.
Conclusion
Choosing the right labeling platform is a strategic decision that can greatly influence your AI project’s success. By focusing on your project’s unique requirements—data type, scalability, quality control, and budget—you can find a platform that ensures high-quality annotations, supports automation, and aligns seamlessly with your workflows.