Introduction
In the rapidly evolving landscape of artificial intelligence (AI) and machine learning (ML), the importance of data annotation cannot be overstated. Data annotation is the process of labeling data to make it understandable for AI and ML models. It serves as the foundation of training datasets, enabling models to learn from annotated examples and make accurate predictions. As businesses increasingly adopt AI-driven solutions, the demand for high-quality data annotation services has surged. Choosing the right data annotation company is critical to the success of AI projects. This comprehensive guide will explore the key factors to consider when selecting a data annotation company, highlight best practices, and provide insights into the leading players in the industry.
Understanding Data Annotation
What is Data Annotation?
Data annotation involves the process of labeling data to provide context and meaning for AI and ML models. This can include annotating images, text, audio, and video data. The labeled data serves as training material for models to learn patterns, relationships, and insights.
Types of Data Annotation
Image Annotation: Labeling objects, regions, or attributes within images. This is crucial for computer vision applications like object detection, facial recognition, and medical imaging.
Text Annotation: Involves tagging parts of speech, named entities, sentiment, and more. This enhances natural language processing (NLP) applications such as chatbots, sentiment analysis, and language translation.
Audio Annotation: Includes transcription, speaker identification, and emotion recognition. It is essential for speech-to-text applications and voice recognition systems.
Video Annotation: Covers activities like object tracking, action recognition, and event detection, facilitating video analysis for surveillance, entertainment, and autonomous driving.
Why Choosing the Right Data Annotation Company Matters
Impact on Model Accuracy
The quality of data annotations directly impacts the accuracy and reliability of AI models. Poorly annotated data can lead to incorrect predictions, reduced model performance, and potential biases.
Scalability and Efficiency
The ability to scale annotation services according to project needs and handle large volumes of data efficiently is crucial for growing businesses and extensive datasets.
Compliance and Security
Adherence to regulatory standards (e.g., GDPR, HIPAA) is critical, especially when dealing with sensitive data in industries like healthcare and finance.
Cost-Effectiveness
Choosing a cost-effective data annotation partner ensures that projects stay within budget without compromising on quality.
Technological Capabilities
Advanced annotation tools, AI-assisted processes, and integration capabilities with AI frameworks contribute to efficient and effective data annotation solutions.
Key Factors to Consider When Choosing a Data Annotation Company
Accuracy and Quality Control
Quality Assurance Processes
Look for companies that have robust quality control measures in place, including multi-layered review processes, automated checks, and regular audits.
Annotation Accuracy
High accuracy rates are essential for the success of AI models. Inquire about the company’s accuracy benchmarks and how they achieve them.
Expertise and Experience
Domain Expertise
Consider companies with experience in your specific industry or application area. Domain expertise ensures that annotators understand the nuances of the data they are labeling.
Track Record
Review the company’s portfolio, case studies, and client testimonials to assess their experience and success in delivering high-quality annotation services.
Scalability and Flexibility
Scalability
Ensure the company can scale their services to meet your project’s needs, whether it involves handling large volumes of data or accommodating fluctuating workloads.
Flexibility
Look for providers that offer flexible annotation workflows, allowing for customization and adjustments based on project requirements.
Compliance and Security
Regulatory Compliance
Verify that the company adheres to relevant data protection regulations such as GDPR, HIPAA, and CCPA.
Data Security
Assess the company’s data security measures, including encryption, secure data transfer protocols, and access controls to protect sensitive information.
Technological Capabilities
Annotation Tools
Advanced annotation tools and platforms can significantly enhance the efficiency and accuracy of the annotation process. Inquire about the tools the company uses and their features.
AI-Assisted Annotation
Companies that leverage AI-assisted annotation can expedite the annotation process and improve accuracy through automation.
Cost-Effectiveness
Pricing Models
Compare pricing models and structures to ensure they align with your budget. Look for transparent pricing without hidden fees.
Value for Money
Assess the overall value provided by the company, considering factors such as quality, turnaround time, and customer support.
Customer Support and Collaboration
Communication
Effective communication is crucial for successful collaboration. Ensure the company provides regular updates, responsive support, and open channels for feedback.
Project Management
A dedicated project manager can streamline the annotation process, coordinate tasks, and address any issues that arise.
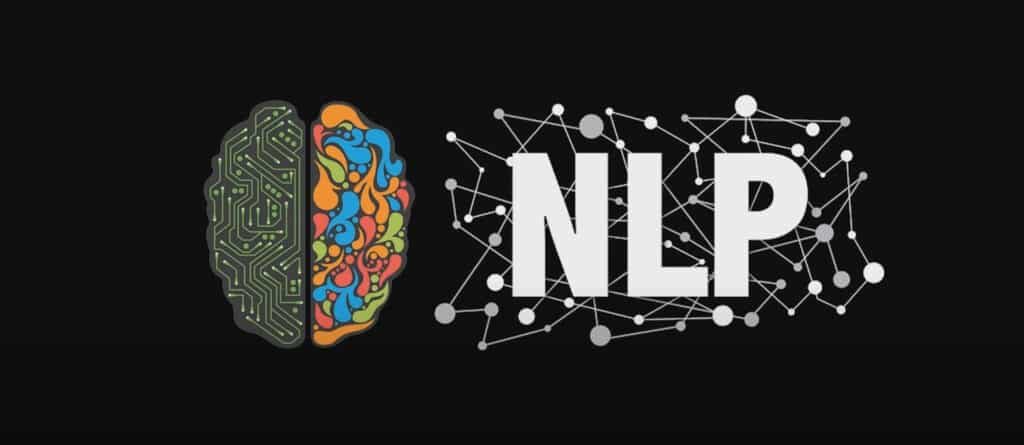
Best Practices for Selecting a Data Annotation Company
Define Your Requirements
Clearly outline your project’s requirements, including the type of data annotation needed, volume of data, accuracy expectations, and timeline. This will help you communicate effectively with potential providers and evaluate their capabilities.
Conduct Thorough Research
Research potential data annotation companies, considering factors such as their expertise, experience, and reputation in the industry. Review case studies, client testimonials, and any available performance metrics.
Request Samples and Demos
Request sample annotations or demos to assess the quality of work provided by the company. This will give you a firsthand look at their annotation accuracy, attention to detail, and overall quality.
Evaluate Technological Capabilities
Inquire about the tools and technologies used by the company. Advanced annotation tools and AI-assisted processes can significantly enhance efficiency and accuracy.
Assess Quality Control Measures
Quality control is critical to ensuring high-quality annotations. Inquire about the company’s quality assurance processes, including multi-layered reviews, automated checks, and regular audits.
Consider Scalability and Flexibility
Ensure the company can scale their services to meet your project’s needs and accommodate any changes in requirements. Flexibility in annotation workflows is also important for customization.
Verify Compliance and Security
Check that the company adheres to relevant data protection regulations and has robust data security measures in place. This is especially important when dealing with sensitive data.
Compare Pricing Models
Evaluate pricing models and structures to ensure they align with your budget. Look for transparent pricing without hidden fees and consider the overall value provided by the company.
Prioritize Customer Support and Collaboration
Effective communication and collaboration are essential for successful data annotation projects. Ensure the company provides regular updates, responsive support, and open channels for feedback.
Leading Data Annotation Companies and Their Best Solutions
SO Development:
SO Development is emerging as a prominent player in the data annotation industry, known for its specialization in healthcare AI, genomic data annotation, and comprehensive annotation solutions.
Strengths: Focus on accuracy and quality, scalability for healthcare applications, compliance with regulatory standards, and customer-centric approach.
Unique Offerings: Expertise in healthcare data annotation, AI-assisted annotation tools, flexible pricing models, and commitment to technological innovation.
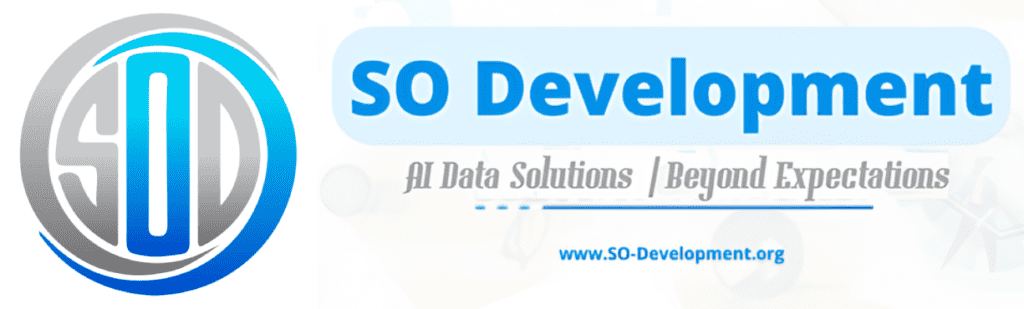
Appen
Appen is a global leader in data annotation and AI training data services. They provide comprehensive solutions for speech, image, text, and video annotations, leveraging a global crowd of annotators.
Strengths: Multilingual expertise, cultural nuances, and compliance with international data protection regulations.
Unique Offerings: Specialized annotation services, linguistic validation, and scalable workforce solutions for large-scale projects.
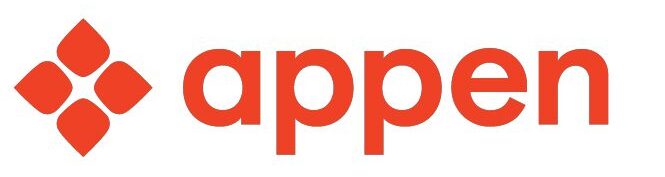
Lionbridge AI
Lionbridge AI offers a wide range of data annotation services, including image, video, text, and audio annotations. They leverage a global crowd of annotators and specialize in linguistic nuances for accurate annotations.
Strengths: Global reach, linguistic expertise, and scalable solutions for diverse datasets and industry-specific requirements.
Unique Offerings: Multilingual capabilities, cultural understanding, and domain-specific annotation expertise.

Scale AI
Scale AI specializes in providing high-quality annotations for computer vision and natural language processing applications. They offer scalable annotation services with a focus on precision and accuracy.
Strengths: Expert annotators, advanced quality assurance, and real-time integration capabilities with AI models.
Unique Offerings: Customizable annotation workflows, tailored solutions for complex datasets, and commitment to delivering accurate annotations.
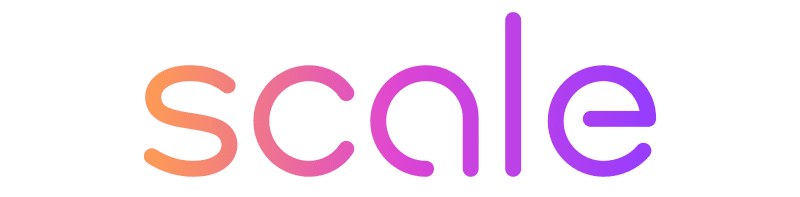
iMerit
Mighty AI specializes in image and video annotation for applications such as autonomous vehicles and robotics. They provide precise annotations to train AI models for object detection and classification.
Strengths: Expertise in computer vision, quality assurance processes, and scalable annotation solutions.
Unique Offerings: Advanced annotation tools, custom annotation workflows, and integration with AI development platforms.
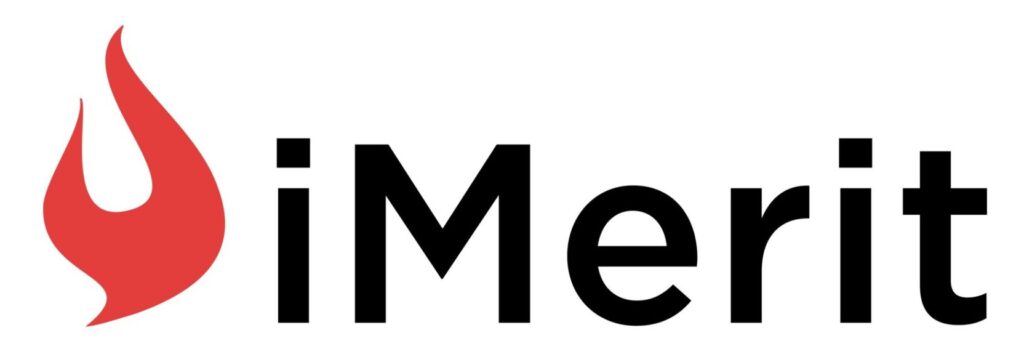
CloudFactory
CloudFactory provides managed workforce solutions for data annotation and data enrichment, specializing in image, video, and text annotations. They emphasize scalability, customization, and secure data handling practices.
Strengths: Managed services, flexible deployment options, and quality assurance processes ensuring accurate annotations.
Unique Offerings: Integration with AI workflows, customized annotation workflows, and robust project management capabilities.
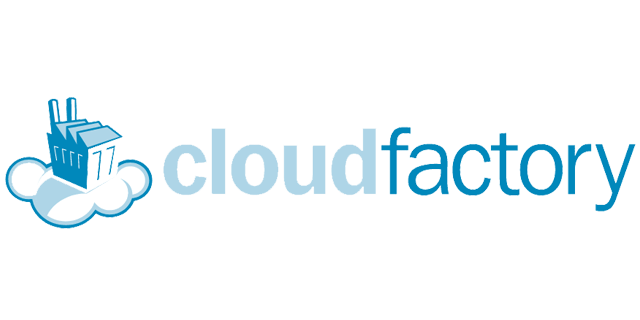
Conclusion
Choosing the right data annotation company is a critical decision that can significantly impact the success of AI and ML projects. By considering factors such as accuracy, expertise, scalability, compliance, technology, cost-effectiveness, and customer support, businesses can make informed choices that align with their project needs and goals. Leading companies like Labelbox, Scale AI, Appen, CloudFactory, Lionbridge AI, Mighty AI, and SO Development offer a range of solutions tailored to diverse industry requirements. Their commitment to quality, innovation, and customer satisfaction makes them reliable partners in the journey towards successful AI implementation.